On January 1st, 2018, I made predictions about self driving cars, Artificial Intelligence, machine learning, and robotics, and about progress in the space industry. Those predictions had dates attached to them for 32 years up through January 1st, 2050.
As part of self certifying the seriousness of my predictions I promised to review them, as made on January 1st, 2018, every following January 1st for 32 years, the span of the predictions, to see how accurate they were. This is my fifth annual review and self appraisal, following those of 2019, 2020, 2021, and 2022. I am over a seventh of the way there! Sometimes I throw in a new side prediction in these review notes.
I made my predictions because at the time, just like now, I saw an immense amount of hype about these three topics, and the general press and public drawing conclusions about all sorts of things they feared (e.g., truck driving jobs about to disappear, all manual labor of humans about to disappear) or desired (e.g., safe roads about to come into existence, a safe haven for humans on Mars about to start developing) being imminent. My predictions, with dates attached to them, were meant to slow down those expectations, and inject some reality into what I saw as irrational exuberance.
I was accused of being a pessimist, but I viewed what I was saying as being a realist. In the last couple of years I have started to think that I too, reacted to all the hype, and was overly optimistic in some of my predictions. My current belief is that things will go, overall, even slower than I thought five years ago. That is not to say that there has not been great progress in all three fields, but it has not been as overwhelmingly inevitable as the tech zeitgeist thought on January 1st, 2018.
UPDATE of 2019’s Explanation of Annotations
As I said in 2018, I am not going to edit my original post, linked above, at all, even though I see there are a few typos still lurking in it. Instead I have copied the three tables of predictions below from 2022’s update post, and have simply added comments to the fourth columns of the three tables. I also highlight dates in column two where the time they refer to has arrived.
I tag each comment in the fourth column with a Cyan (#00ffff) colored date tag in the form yyyymmdd such as 20190603 for June 3rd, 2019. As in 2022 I have highlighted the new text put in for the current year in LemonChiffon (#fffacd) so that it is easy to pick out this year’s updates. There are 15 such updates this year in the tables below.
The entries that I put in the second column of each table, titled “Date” in each case, back on January 1st of 2018, have the following forms:
NIML meaning “Not In My Lifetime, i.e., not until beyond December 31st, 2049, the last day of the first half of the 21st century.
NET some date, meaning “No Earlier Than” that date.
BY some date, meaning “By” that date.
Sometimes I gave both a NET and a BY for a single prediction, establishing a window in which I believe it will happen.
For now I am coloring those statements when it can be determined already whether I was correct or not.
I have started using LawnGreen (#7cfc00) for those predictions which were entirely accurate. For instance a BY 2018 can be colored green if the predicted thing did happen in 2018, as can a NET 2019 if it did not happen in 2018 or earlier. There are 14 predictions now colored green, including 4 new ones this year.
I will color dates Tomato (#ff6347) if I was too pessimistic about them. There is one Tomato, with no new ones this year. If something happens that I said NIML, for instance, then it would go Tomato, or if in 2020 something already had happened that I said NET 2021, then that too would have gone Tomato.
If I was too optimistic about something, e.g., if I had said BY 2018, and it hadn’t yet happened, then I would color it DeepSkyBlue (#00bfff). The first of these appeared this year. And eventually if there are NETs that went green, but years later have still not come to pass I may start coloring them LightSkyBlue (#87cefa). I did that below for one prediction in self driving cars last year.
In summary then: Green splashes mean I got things exactly right. Red means provably wrong and that I was too pessimistic. And blueness will mean that I was overly optimistic.
Self Driving Cars
ot the predictions were made and the years in blue were the originally predicted times when the capability or deployment would happen. (The orange arrows indicate that I later found revised dates from the same sources.) When a blue year passes without the prediction having been fulfilled I color it pinkish. No new pink this year, but as before not a single one of these predictions has come to fruition, so far not ever, not just that they have happened, but later. These predictions were part of what made me make my predictions back in 2018 to temper them with reality.
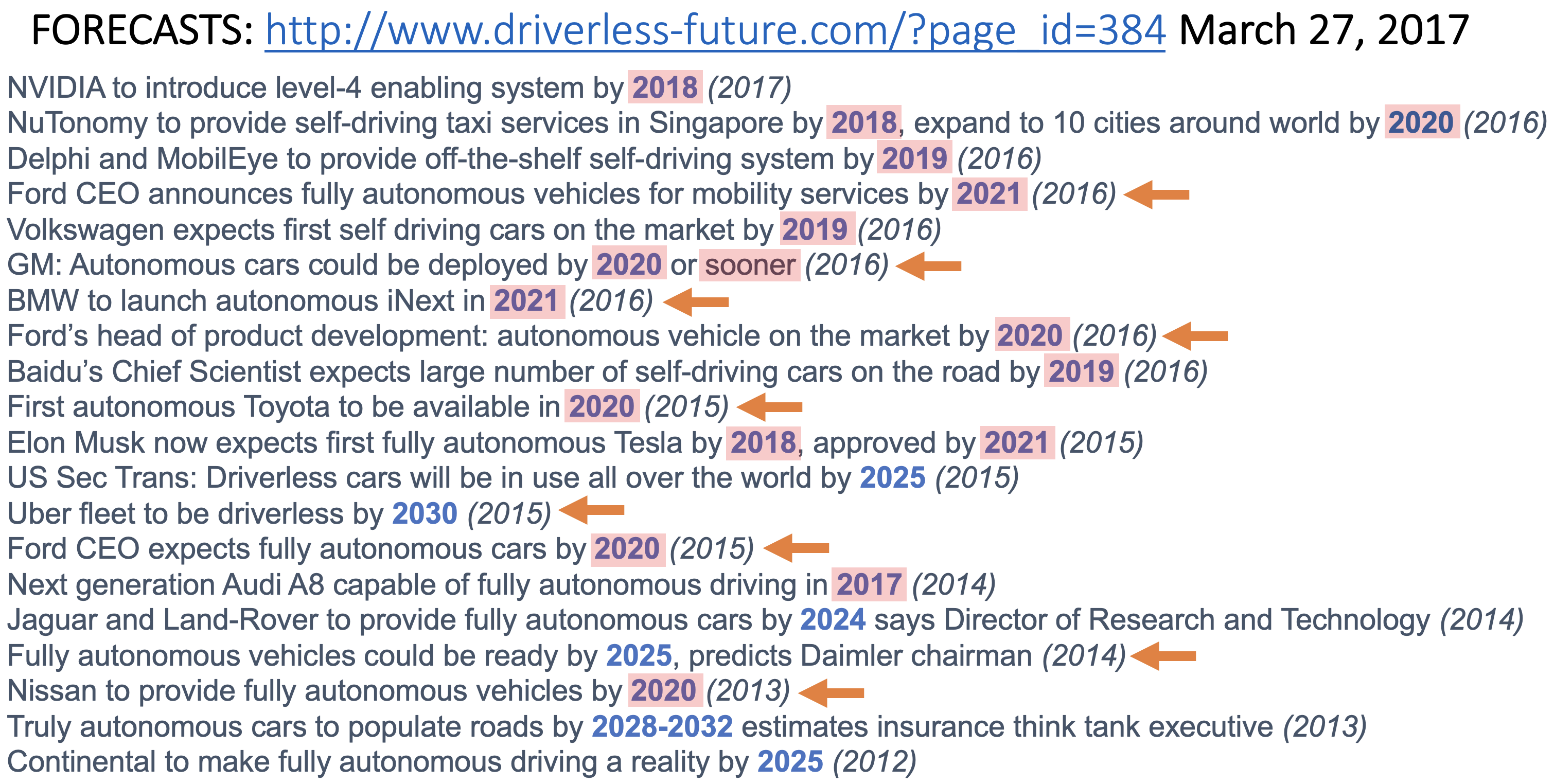
At the end of 2024 and 2025, the next years that show up in blue here, I fully expect to be able to color them pink.
But, I am no longer alone. In the last year there has been a significant shift, and belief in self driving cars being imminent and/or common has started to have its wheels fall off.
Bloomberg complained that despite one hundred billion dollars having been spent on their development self driving care are going nowhere.
More importantly various efforts are being shut down, despite billions of dollars of sunk cost. After spending $3.6B Ford and VW shut down their joint venture, Argo, as the story says, a self-driving road to nowhere. As this story says, Ford thought they could bring the technology to market in 2021 (as reported in the graphic above), but they think maintaining 2,000 employees in search of this opportunity is not the best way to serve their customers.
Many other car companies have pulled back from saying they are working on driverless software, or Level 4. Toyota and Mercedes are two such companies, where they expect a driver to be in the loop, and are not trying to remove them.
Meanwhile the heat is on Tesla for naming their $15,000 software Full Self Driving (FSD), and the state of California is fighting them in court.
See this very careful review of Telsa FSD driving in a Jacksonville, FL, neighborhood, with the car’s owner, airline pilot Chuck Cook. He is a true fan of Tesla and FSD, but tests it rigorously, so much so that the CEO of Tesla has sent engineers to work with him and tune up FSD for his neighborhood. As this review shows it is still not reliable in many circumstances. If it is not reliable sometimes then the human has to be paying attention at all times and so it is a long way from actually being FSD. People will make excuses for it, but that won’t work with normal people, like me. Paying $15,000 for software which doesn’t do its primary job, namely giving the human full confidence, is not a winning business strategy once you get out of the techy bubble.
Oh, and by the way, the “one million robo-taxis” on the road by 2020, promised by the CEO of Tesla in early 2019 have still not panned out. I believe the actual number is still solidly zero.
Other parts of the great self driving experiment have also proved to be too expensive. This year Amazon shut down its last mile autonomous delivery service project, where the idea was that driverless vehicles would get their packages directly to houses. Too hard for now.
But I Did Take Self-Driving Taxi Services in 2022
Back in May, on this blog, I reported my experience taking a truly driverless taxi service three times in one evening in San Francisco. It was with Cruise (owned my GM) who now charge money for this service that runs on good weather nights from around 10:30pm to 5:00am. These are the hours of least traffic in San Francisco. It does not operate in all parts of the city, especially not the crowded congested parts.
Here is the brief summary of my experience.. The first ride was to be about 20 blocks due south of my house. The Cruise vehicle did not come to my house but told me to go to another street at the end of my block. My block is quite difficult to drive on for a number of reasons and I never see any of Cruise, Waymo, or Zoox vehicles collecting data on it, though they can all be seen just a block away.
Rather than heading due south the Cruise vehicle diverted 10 blocks west and back, most probably to avoid areas with traffic. The few times it was in traffic it reminded me of when I taught my kids to drive with heavy unnecessary breaking when other cars were nearby. It also avoid unprotected left turns and preferred to loop around through a series of three right turns.
On my third ride it elected to pick me up on the other side of the street than where I had requested it, right in front of construction that forced me to walk out into active lanes in order to get in. No human taxi driver would have ignored my waves and gestures to be picked up at a safe spot thirty feet further along the road.
My complete report includes many other details. Functionally it worked, but it did so by driving slowly and avoiding any signs of congestion. The result is that it was slower by a factor of two over any human operated ride hailing service. That might work for select geographies, but it is not going to compete with human operated systems for quite a while.
That said, I must congratulate Cruise on getting it so far along. This is much more impressive and real than any other company’s attempts at deployment. But as far as I can tell it is only about 32 vehicles, and Cruise is losing $5M per day. This is decades away from profitability.
Here is part of a comment on my blog post by a Glenn Mercer, who I don’t think that I know (apologies Glenn, if I do or should!!) [Chorizo is the name of a car that took me on two of my three rides.]:
Your experience seemed similar: your rides seemed to “try” (sorry about imputing agency here) to be safe by avoiding tricky situations. It would be underwhelming that if all AVs delivered to us was confirmation of the already-known fact that we can cut fatalities in the USA by obeying traffic laws, driving cautiously, not drinking, etc. Of course ANY fatality avoided is a good thing… but it is a little underwhelming (from the perspective of a fan of all things sci-fi) that we may avoid them not because some sentient AI detected via satellite imagery a hurtling SUV 3 blocks away and calculated its trajectory in real time to induce in Chorizo a spectacular life-saving 3-point turn… Rather, that just making sure Chorizo stopped at the stop sign and “looked both ways” may be all that we needed. Ah, well.
I think this is a great comment. It fits well with my prediction that no self driving car is going to be faced with an instance of the trolley problem until beyond 2050. My advice on the so called “trolley problem” is to just stomp on the damn brakes. That fits with Glenn’s recommendation above to just be consistently careful, and you’ll do a whole lot better than most humans. This may well be achievable.
Prediction
[Self Driving Cars] | Date | 2018 Comments | Updates |
A flying car can be purchased by any US resident if they have enough money. | NET 2036 | There is a real possibility that this will not happen at all by 2050.
| 20230101 There is currently frothy hype about coming flying electric taxi services. See the main text for why I think this hype is overblown. And note that these eVTOL taxis are not at all what people used to mean (just five years ago) when they said "flying cars". |
Flying cars reach 0.01% of US total cars. | NET 2042 | That would be about 26,000 flying cars given today's total. | |
Flying cars reach 0.1% of US total cars. | NIML | | |
First dedicated lane where only cars in truly driverless mode are allowed on a public freeway.
| NET 2021 | This is a bit like current day HOV lanes. My bet is the left most lane on 101 between SF and Silicon Valley (currently largely the domain of speeding Teslas in any case). People will have to have their hands on the wheel until the car is in the dedicated lane. | 20210101 It didn't happen any earlier than 2021, so I was technically correct. But I really thought this was the path to getting autonomous cars on our freeways safely. No one seems to be working on this...
20220101 Perhaps I was projecting my solution to how to get self driving cars to happen sooner than the one for one replacement approach that the Autonomous Vehicle companies have been taking. The left lanes of 101 are being rebuilt at this moment, but only as a toll lane--no special assistance for AVs. I've turned the color on this one to "too optimistic" on my part. |
Such a dedicated lane where the cars communicate and drive with reduced spacing at higher speed than people are allowed to drive | NET 2024 | | |
First driverless "taxi" service in a major US city, with dedicated pick up and drop off points, and restrictions on weather and time of day. | NET 2021 | The pick up and drop off points will not be parking spots, but like bus stops they will be marked and restricted for that purpose only. | 20190101 Although a few such services have been announced every one of them operates with human safety drivers on board. And some operate on a fixed route and so do not count as a "taxi" service--they are shuttle buses. And those that are "taxi" services only let a very small number of carefully pre-approved people use them. We'll have more to argue about when any of these services do truly go driverless. That means no human driver in the vehicle, or even operating it remotely.
20200101
During 2019 Waymo started operating a 'taxi service' in Chandler, Arizona, with no human driver in the vehicles. While this is a big step forward see comments below for why this is not yet a driverless taxi service.
20210101 It wasn't true last year, despite the headlines, and it is still not true. No, not, no.
20220101 It still didn't happen in any meaningful way, even in Chandler. So I can call this prediction as correct, though I now think it will turn out to have been wildly optimistic on my part. 20230101 There was movement here, in that Cruise started a service in San Francisco, for a few hours per night, with about 32 vehicles. I rode it before it was charging money, but it now is doing so. Cruise is still losing $5M per day, however. See the main text for details. |
Such "taxi" services where the cars are also used with drivers at other times and with extended geography, in 10 major US cities | NET 2025 | A key predictor here is when the sensors get cheap enough that using the car with a driver and not using those sensors still makes economic sense. | |
Such "taxi" service as above in 50 of the 100 biggest US cities. | NET 2028 | It will be a very slow start and roll out. The designated pick up and drop off points may be used by multiple vendors, with communication between them in order to schedule cars in and out.
| |
Dedicated driverless package delivery vehicles in very restricted geographies of a major US city. | NET 2023 | The geographies will have to be where the roads are wide enough for other drivers to get around stopped vehicles.
| 20220101 There are no vehicles delivering packages anywhere. There are some food robots on campuses, but nothing close to delivering packages on city streets. I'm not seeing any signs that this will happen in 2022. 20230101 It didn't happen in 2022, so I can call it. It looks unlikely to happen any time soon as some major players working in this area, e.g., Amazon, abandoned their attempts to develop this capability (see the main text). It is much harder than people thought, and large scale good faith attempts to do it have shown that. |
A (profitable) parking garage where certain brands of cars can be left and picked up at the entrance and they will go park themselves in a human free environment. | NET 2023 | The economic incentive is much higher parking density, and it will require communication between the cars and the garage infrastructure. | 20220101 There has not been any visible progress towards this that I can see, so I think my prediction is pretty safe. Again I was perhaps projecting my own thoughts on how to get to anything profitable in the AV space in a reasonable amount of time. 20230101 It didn't happen in 2022, so I can call this prediction as correct. I think Tesla's driving system is probably good enough for their cars to do this, but no one seems to be going after this small change application. |
A driverless "taxi" service in a major US city with arbitrary pick and drop off locations, even in a restricted geographical area.
| NET 2032 | This is what Uber, Lyft, and conventional taxi services can do today. | |
Driverless taxi services operating on all streets in Cambridgeport, MA, and Greenwich Village, NY. | NET 2035 | Unless parking and human drivers are banned from those areas before then. | |
A major city bans parking and cars with drivers from a non-trivial portion of a city so that driverless cars have free reign in that area. | NET 2027
BY 2031 | This will be the starting point for a turning of the tide towards driverless cars. | |
The majority of US cities have the majority of their downtown under such rules. | NET 2045 | | |
Electric cars hit 30% of US car sales. | NET 2027 | | 20230101 I made this prediction five years ago today. If it is accurate we'll know five years from today. When I made the prediction many people on social media said I was way too pessimistic. Looking at the current numbers (see the main text) I think this level of sales could be plausibly reached in one of 2026, 2027, or 2028. Any small perturbance will knock 2026 out of contention. In the worst (for me) case perhaps I was pessimistic in predicting ten years rather than nine for this to happen. |
Electric car sales in the US make up essentially 100% of the sales. | NET 2038
| | |
Individually owned cars can go underground onto a pallet and be whisked underground to another location in a city at more than 100mph. | NIML | There might be some small demonstration projects, but they will be just that, not real, viable mass market services.
| |
First time that a car equipped with some version of a solution for the trolley problem is involved in an accident where it is practically invoked. | NIML | Recall that a variation of this was a key plot aspect in the movie "I, Robot", where a robot had rescued the Will Smith character after a car accident at the expense of letting a young girl die. | |
Other Car Predictions — Electric Cars
In my 2018 predictions I said that electric cars would not make up 30% of US automobile sales until 2027 (and note that the 2027 sales level can only be known sometime in 2028, ten years after my prediction).
This prediction was at odds with the froth about Tesla, and indeed its stock price over the last few years, until Q4 2022, when things changed dramatically. I wondered whether I had been too optimistic.
However, there was a big uptick in electric car sales in the US in Q3 2022, as can be seen by comparing the Blue Book numbers on sales of all vehicles with those of electric vehicles. In Q3 electric vehicle sales were 205,682 (a year on year increase of 68%) compared to a total of 3,418,718 for all vehicle sales (a mere 0.1% year on year increase), making electric sales 6% of all US sales. Clearly something is going on.
If electric sales continue to grow at 68% per year then 2025 would hit 28% of new cars in the US being electric. Even at 50% year over year growth we would get to 30% in 2026, a year earlier than I had predicted. But those are both big ifs, and one quarter of big growth does not a make a trend. We need to see this sustained for a while.
Meanwhile there are forces ready to slow down electric car adoption, including dropping gas prices. Or perhaps if some of the automobile battery plants are delayed for any of a hundred reasons. If everything goes right I think there is a chance of meeting 30% electric vehicles in the US sometime in the 2026 to 2028 period.
Not everyone agrees however, including the leader of the biggest car company in the world. Just this last week Akio Toyoda, CEO of Toyota, has been quoted trying to temper expectations: “Just like the fully autonomous cars that we were all supposed to be driving by now, I think BEVs are just going to take longer to become mainstream than the media would like us to believe.” (Note that he also casually mentions that self driving cars are much harder than everyone thought. Also he calls them Battery Electric Vehicles as Toyota also produces a fuel cell based electric vehicle that consumes hydrogen.)
So…electric car adoption is rising, but the jury is still out on whether we will get to 30% US market penetration by 2027.
Other Car Predictions — Flying Cars
Way back when I made my predictions in 2018 “flying cars” meant cars that could drive on regular roads and then take to the air and fly somewhere. Over the last few years the meaning of “flying cars” has drifted to things that are not cars at all, but rather very light electric vertical take off and landing (eVTOL) flying machines that will be flying taxis whisking people over the crowded freeways to their destinations. A utopian transport structure.
Needless to say there has been froth galore on just how big this industry is going to be and just how soon it is going to happen, and just how big a revolution it will be.
Yeah.
Recently I did an analysis, published on this blog, of a McKinsey report saying all these things. I allowed that perhaps they were 100 times too optimistic (what is a factor of 100 too big as a market analysis between friends, after all), and if we allowed for 400% growth per year to meet that 100th sized market then today there must be seven flights per day of eVTOLs, following a commercial profile and with people onboard. So… there should be videos of such flights, somewhere, right?
No, not anywhere. There are some uncrewed flights, and there are some just a few tens of meters high over water, but none over populated areas and none at the hundreds of meters that will need to be maintained. And the videos on the websites of companies that have taken hundreds of millions of dollars in VC funding show only two meter high crewed hops. And perhaps more tellingly, later in 2022, Larry Page pulled the plug on Kitty Hawk, a company he had been funding for 12 years to develop such capabilities.
One way or another, at scale eVTOL taxis are not happening soon.
Robotics, AI, and Machine Learning
British science fiction and science writer Arthur C. Clarke formulated three adages that have come to be known as Clarke’s three laws. The third of these is:
Any sufficiently advanced technology is indistinguishable from magic.
As I said in my post seven deadly sins of predicting the future of AI.
This is a problem we all have with imagined future technology. If it is far enough away from the technology we have and understand today, then we do not know its limitations. It becomes indistinguishable from magic.
When a technology passes that magic line anything one says about it is no longer falsifiable, because it is magic.
When technology is sufficiently different from what we have experienced to date, we humans can’t make good guesses about its limitations. And so we start imbuing it with magical powers, believing that it is more powerful than anything we have previously imagined, so it becomes at once both incredibly powerful and incredibly dangerous.
A more detailed problem is what I call the performance/competence confusion, also from that seven deadly sins blog post.
We humans have a good mapping for people in understanding what a particular performance by a person in some arena implies about the general competence of that person in that arena. For instance, if a person is able to carry on a conversation about a radiological images that they are looking it is a safe bet that that person would be able to look out of the window and tell you what the weather is out there. And even more, tell you the sorts of weather that one typically sees over the period of a year in that same location (e.g., “no snow here, but lots of rain in February and March”).
The same can not be said for our AI systems. Performance on some particular task in no indicator of any human like general competence in related areas.
In recent years we saw this with Deep Learning (and Reinforcement Learning for that matter, though the details of the Alpha game players were much more complex than most people understood). DL was supposed to make both radiologists and truck drivers redundant in just a few years. Instead we have a shortage of people in each of these occupations. On the other hand DL has given both those professions valuable tools.
This past year has seen Dall-E 2, the latest image from natural language system, and ChatGPT, the latest large language model. When people see cherry picked examples from these they think that general machine intelligence, with both promise and danger, is just around the corner.
There is a veritable cottage industry on social media with two sides; one gushes over virtuoso performances of these systems, perhaps cherry picked, and the other shows how incompetent they are at very simple things, again cherry picked.
The problem is that as a user you don’t know in advance what you are going to get. So humans still need their hands on the information space steering wheel at all times in order not to end up with a total wreck of an outcome in any applications built on these systems.
Vice Admiral Joe Dyer, former chief test pilot of the US Navy, once reminded me that nothing is ever as good as it first seems, nor as bad. That is an incredibly helpful adage.
Calm down people. We neither have super powerful AI around the corner, nor the end of the world caused by AI about to come down upon us.
And that pretty much sums up where AI and Machine Learning have gone this year. Lots of froth and not much actual deployed new hard core reliable technology.
Prediction
[AI and ML] | Date | 2018 Comments | Updates |
Academic rumblings about the limits of Deep Learning | BY 2017 | Oh, this is already happening... the pace will pick up. | 20190101 There were plenty of papers published on limits of Deep Learning. I've provided links to some right below this table. 20200101
Go back to last year's update to see them. |
The technical press starts reporting about limits of Deep Learning, and limits of reinforcement learning of game play. | BY 2018 | | 20190101 Likewise some technical press stories are linked below. 20200101
Go back to last year's update to see them. |
The popular press starts having stories that the era of Deep Learning is over. | BY 2020 | | 20200101 We are seeing more and more opinion pieces by non-reporters saying this, but still not quite at the tipping point where reporters come at and say it. Axios and WIRED are getting close.
20210101 While hype remains the major topic of AI stories in the popular press, some outlets, such as The Economist (see after the table) have come to terms with DL having been oversold. So we are there. |
VCs figure out that for an investment to pay off there needs to be something more than "X + Deep Learning". | NET 2021 | I am being a little cynical here, and of course there will be no way to know when things change exactly. | 20210101 This is the first place where I am admitting that I was too pessimistic. I wrote this prediction when I was frustrated with VCs and let that frustration get the better of me. That was stupid of me. Many VCs figured out the hype and are focusing on fundamentals. That is good for the field, and the world! |
Emergence of the generally agreed upon "next big thing" in AI beyond deep learning. | NET 2023 BY 2027 | Whatever this turns out to be, it will be something that someone is already working on, and there are already published papers about it. There will be many claims on this title earlier than 2023, but none of them will pan out. | 20210101 So far I don't see any real candidates for this, but that is OK. It may take a while. What we are seeing is new understanding of capabilities missing from the current most popular parts of AI. They include "common sense" and "attention". Progress on these will probably come from new techniques, and perhaps one of those techniques will turn out to be the new "big thing" in AI.
20220101 There are two or three candidates bubbling up, but all coming out of the now tradition of deep learning. Still no completely new "next big thing". 20230101 Lots of people seem to be converging on "neuro-symbolic", as it addresses things missing in large language models. |
The press, and researchers, generally mature beyond the so-called "Turing Test" and Asimov's three laws as valid measures of progress in AI and ML. | NET 2022 | I wish, I really wish. | 20220101 I think we are right on the cusp of this happening. The serious tech press has run stories in 2021 about the need to update, but both the Turing Test and Asimov's Laws still show up in the popular press. 2022 will be the switchover year. [Am I guilty of confirmation bias in my analysis of whether it is just about to happen?] 20230101 The Turing Test was missing from all the breathless press coverage of ChatGPT and friends in 2022. Their performance, though not consistent, pushes way past the old comparisons. |
Dexterous robot hands generally available. | NET 2030
BY 2040 (I hope!) | Despite some impressive lab demonstrations we have not actually seen any improvement in widely deployed robotic hands or end effectors in the last 40 years. | |
A robot that can navigate around just about any US home, with its steps, its clutter, its narrow pathways between furniture, etc. | Lab demo: NET 2026
Expensive product: NET 2030
Affordable product: NET 2035 | What is easy for humans is still very, very hard for robots. | 20220101 There was some impressive progress in this direction this year with the Amazon's release of Astro. A necessary step towards these much harder goals. See the main text. 20230101 Astro does not seem to have taken off, and there are no new demos of it for public consumption. We may have to wait for someone else to pick up the mantle. |
A robot that can provide physical assistance to the elderly over multiple tasks (e.g., getting into and out of bed, washing, using the toilet, etc.) rather than just a point solution. | NET 2028 | There may be point solution robots before that. But soon the houses of the elderly will be cluttered with too many robots. | |
A robot that can carry out the last 10 yards of delivery, getting from a vehicle into a house and putting the package inside the front door. | Lab demo: NET 2025
Deployed systems: NET 2028
| | |
A conversational agent that both carries long term context, and does not easily fall into recognizable and repeated patterns. | Lab demo: NET 2023 Deployed systems: 2025 | Deployment platforms already exist (e.g., Google Home and Amazon Echo) so it will be a fast track from lab demo to wide spread deployment. | 20230101 Despite the performance of ChatGPT and other natural language transformer models, no one seems to have connected them to spoken language systems, nor have their text versions demonstrated any understanding of interactional context over periods of time with individuals. Perhaps people have tried but the shortcomings of the systems dominate in this mode. |
An AI system with an ongoing existence (no day is the repeat of another day as it currently is for all AI systems) at the level of a mouse. | NET 2030 | I will need a whole new blog post to explain this... | |
A robot that seems as intelligent, as attentive, and as faithful, as a dog. | NET 2048 | This is so much harder than most people imagine it to be--many think we are already there; I say we are not at all there. | |
A robot that has any real idea about its own existence, or the existence of humans in the way that a six year old understands humans. | NIML | | |
The Next Big Thing in AI
Both Dall-E 2 and ChatGPT have a lot more mechanism in them than just plan back propagation deep neural networks. To make Robotics or AI systems do stuff we always need active mechanism besides any sort of learning algorithm.
In the old days of AI we built active mechanisms with symbols, using techniques known as Symbolic Artificial Intelligence. Symbols are atomic computational objects which stand in place of something out in the physical world.
Over the last year there has been a new term making the rounds, “neuro-symbolic” or “neurosymbolic”, as a way of merging the deep learning revolution with symbolic artificial intelligence. Although everyone is very protective of their unique angle on this merger it seems that lots of people from all over the field of Artificial Intelligence believe there may be something there. One might even classify the two media darlings above as instances of neurosymbolic AI.
In Q3 of 2022 a new journal Neurosymbolic Artificial Intelligence showed up. It has an editorial board with representation from first rate institutions across the globe.
Note that the wikipedia page on symbolic AI, referenced above, was edited in August 2022 to include the term “neuro-symbolic” for the first time. Whether neuro-symbolic becomes a real dominant approach is still up in the air. But it has a whiff of possibility about it.
[[I don’t happen to think that neuro-symbolic will past the test of time, but it will lead to more short term progress. I’ll write a longer post on why I think it still misses out on being a solid foundation for artificially intelligent machines over the next few centuries.]]
Space
The biggest story of the last year is the lack of any further flights of SpaceX’s Starship. Late in 2021 the CEO was talking about getting to a flight every two weeks by the end of 2022. But none, nada, zippo. Normally that might be considered an unbelievable difference in what was promised and what was delivered. But these days CEOs of car companies, social media companies, and yes space transportation companies, have been known to repeatedly make outrageous promises that have nothing to do with reality.
In the case of SpaceX this may be problematic, as the US return to the Moon is premised upon SpaceX delivering. If the government can’t believe the CEO’s promises that is going to make policy decisions and letting contracts very difficult.
NASA has had some success (un-crewed Artemis) towards lunar goals, and Blue Origin has finally delivered engines for a large booster built by others.
SpaceX itself has had a banner year with its Falcon 9 booster with 60 successful launches (plus one Falcon Heavy launch); probably the last time we had a launch rate that large was the V2 in the final year of the Second World War (though the V2 rate was about 50 times higher and its success rate was much lower than that of SpaceX).
Space tourism had a great 2021 but not such a big year in 2022. It is still not a large scale business and likely won’t be for a while, if ever.
Summary: conventional stuff is going great, new stuff is hard and only limping along.
Space Tourism Sub-Orbital
In July 2020 Virgin Galactic had their first suborbital flight which took non-flight crew along, including Richard Branson the founder. It was expected that this would mark the start of their commercial operations but there have been zero further flights of any sort since then with many reasons for the delays.
In 2022 Blue Origin had three flights with people aboard for a total of 18 people. At most 14 of these paid for their tickets while the others were an employee of Blue Origin, or had been selected by other organizations, so they essentially won their seats.
All six crewed Blue Origin flights to date have shared the same hardware including New Shepard 4 as the booster. In September 2022, a month after the sixth crewed flight, an older booster, New Shepard 3, failed during ascent and the flight was aborted and the un-crewed capsule landed safely. There were no further crewed flights for the rest of the year.
In summary crewed sub-orbital flights were down from 2021, and there has been no measurable uptick in paying passengers. These flights are not really taking off at scale.
Space Tourism Orbital
There had been eight non-governmental paid orbital flights up through 2009, all on Russia’s Soyuz. Then none until the last four months of 2021 when there were three; two on Soyuz to the International Space Station and one in a Crew Dragon on a SpaceX Falcon 9 that was self sustaining with no docking in space. A total of eight non-professionals flew to space on those three missions.
This sudden burst of activity made it look like perhaps things might really be taking off, so to speak. But in 2022 there was only one paid orbital flight, a SpaceX Dragon to the International Space Station, carrying three non-professionals and one former NASA Astronaut working for the company that organized the flight and purchased it from SpaceX.
The space tourism business is still at the sputtering initial steps phase.
SpaceX Falcon 9
SpaceX had a spectacular year with its Falcon 9 having 60 successful launches, with no launch failures and all boosters that were intended to return to Earth successfully doing so. It also had its first Falcon Heavy launch since 2019. As with all four Falcon Heavy launches this was successful and the two side boosters landed back at the launch site as expected.
Prediction
[Space] | Date | 2018 Comments | Updates |
Next launch of people (test pilots/engineers) on a sub-orbital flight by a private company. | BY 2018 | | 20190101 Virgin Galactic did this on December 13, 2018.
20200101 On February 22, 2019, Virgin Galactic had their second flight, this time with three humans on board, to space of their current vehicle. As far as I can tell that is the only sub-orbital flight of humans in 2019. Blue Origin's new Shepard flew three times in 2019, but with no people aboard as in all its flights so far.
20210101 There were no manned suborbital flights in 2020. |
A few handfuls of customers, paying for those flights. | NET 2020 | | 20210101 Things will have to speed up if this is going to happen even in 2021. I may have been too optimistic.
20220101 It looks like six people paid in 2021 so still not a few handfuls. Plausible that it happens in 2022. 20230101 There were three such flights in 2022, with perhaps 14 of the 18 passengers paying. Not quite there yet. |
A regular sub weekly cadence of such flights. | NET 2022 BY 2026 | | 20220101 Given that 2021 only saw four such flights, it is unlikely that this will be achieved in 2022. 20230101 Only three flights total in 2022. A long way to go to get to sub weekly flights. |
Regular paying customer orbital flights. | NET 2027 | Russia offered paid flights to the ISS, but there were only 8 such flights (7 different tourists). They are now suspended indefinitely. | 20220101 We went from zero paid orbital flights since 2009 to three in the last four months of 2021, so definitely an uptick in activity. 20230101 Not so quick! Only one paid flight in 2022. |
Next launch of people into orbit on a US booster. | NET 2019 BY 2021 BY 2022 (2 different companies)
| Current schedule says 2018. | 20190101 It didn't happen in 2018. Now both SpaceX and Boeing say they will do it in 2019.
20200101 Both Boeing and SpaceX had major failures with their systems during 2019, though no humans were aboard in either case. So this goal was not achieved in 2019. Both companies are optimistic of getting it done in 2020, as they were for 2019. I'm sure it will happen eventually for both companies. 20200530 SpaceX did it in 2020, so the first company got there within my window, but two years later than they predicted. There is a real risk that Boeing will not make it in 2021, but I think there is still a strong chance that they will by 2022.
20220101 Boeing had another big failure in 2021 and now 2022 is looking unlikely. 20230101 Boeing made up some ground in 2022, but my 2022 prediction was too optimistic. |
Two paying customers go on a loop around the Moon, launch on Falcon Heavy. | NET 2020 | The most recent prediction has been 4th quarter 2018. That is not going to happen. | 20190101 I'm calling this one now as SpaceX has revised their plans from a Falcon Heavy to their still developing BFR (or whatever it gets called), and predict 2023. I.e., it has slipped 5 years in the last year.
20220101 With Starship not yet having launched a first stage 2023 is starting to look unlikely, as one would expect the paying customer (Yusaku Maezawa, who just went to the ISS on a Soyuz last month) would want to see a successful re-entry from a Moon return before going himself. That is a lot of test program to get to there from here in under two years. 20230101 With zero 2022 launch activity of Starship this means it couldn't possibly happen until 2024. |
Land cargo on Mars for humans to use at a later date
| NET 2026 | SpaceX has said by 2022. I think 2026 is optimistic but it might be pushed to happen as a statement that it can be done, rather than for an pressing practical reason. | 20230101 The CEO of SpaceX has a pattern of over-optimistic time frame predictions . This did not happen in 2022 as he predicted. I'm now thinking that my 2026 prediction is way over optimistic, as the only current plan is to use Starship to achieve this. |
Humans on Mars make use of cargo previously landed there. | NET 2032 | Sorry, it is just going to take longer than every one expects. | |
First "permanent" human colony on Mars. | NET 2036 | It will be magical for the human race if this happens by then. It will truly inspire us all.
| |
Point to point transport on Earth in an hour or so (using a BF rocket). | NIML | This will not happen without some major new breakthrough of which we currently have no inkling.
| |
Regular service of Hyperloop between two cities. | NIML | I can't help but be reminded of when Chuck Yeager described the Mercury program as "Spam in a can".
| |
Boeing’s Woes
The Boeing Starliner is a capsule to be used by NASA on a similar commercial basis that NASA uses Crew Dragon from SpaceX. Originally the development of the two systems was neck and neck, but both were delayed beyond original expectations and Boeing’s Starliner fell behind Crew Dragon.
The first un-crewed flight was in December 2019 and there were many serious problems revealed. Boeing decided that it needed to re-fly that mission before putting NASA astronauts aboard and there were repeated delays with the second flight finally happening in May of 2022, where it autonomously docked with the International Space Station and then safely returned to a splashdown on Earth.
The first crewed flight is currently scheduled for April 2023, many years later than the original expectation.
Artemis
NASA successfully flew its Artemis 1 mission, on NASA’s new Space Launch System, and the Orion Capsule, in November and December of 2022. It flew to the Moon and and went into a very elliptical orbit, finally returned to a safe splash down on Earth after 25 days.
The Artemis 2 mission will take astronauts to lunar orbit in May 2024, to be followed by Artemis 3 landing two astronauts on the Moon some time in 2025. There are a total of ten crewed Artemis missions currently planned, out to 2034, with missions 3 through 11 all landing astronauts on the Moon, sometimes for many months at a time. All astronauts will leave Earth on NASA’s Space Launch System. Lunar landings for missions 3 and 4 are currently scheduled to use SpaceX’s Starship. See below. SpaceX Falcon Heavy (triple Falcon 9 boosters) will take hardware to Lunar orbit for Gateway, a small space station.
Starship
Starship is SpaceX’s intended workhorse to replace both Falcon 9 and Falcon Heavy.
It is two stages, both of which are intended to return to Earth. The second stage had many test flights up through early 2021, and after a number of prototypes being destroyed in explosions one successfully landed back at its launch site in Texas. None of these flights left the atmosphere so the heat shields have not yet been tested.
The first stage is massive, with 37 Raptor engines that are a generation later than the Merlins used on Falcon 9. So far it has not flown, nor has it successfully lit as many as 30 of its engines in a ground test.
Over US Thanksgiving in 2021 the CEO of SpaceX urged his workers to abandon their families and come in to work to boost the production rate of these engines. In his email he said:
What it comes down to is that we face genuine risk of bankruptcy if we cannot achieve a Starship flight rate of at least once every two weeks next year.
“Next year” would be 2022. There have been zero test flights of either the second stage or the first stage and it is the end of 2022. The first stage has never flown at all. Not even powered up all its engines. The first stage is much more complex than any previous booster ever built. It is not flying once every two weeks. Does that mean SpaceX is in danger of bankruptcy? Or was the SpaceX CEO using hyperbole to extract work from his employees?
Testing of the Raptor engines continues at the McGregor launch site and sometimes they blow up (most recently on Dec 21st, 2022)–they may well be being tested to destruction when that happens.
I am concerned that Starship will not be ready for the proposed 2025 Lunar Landing of astronauts and that will really delay humankind’s return the to Moon.
Blue Origin’s Orbital Class Rockets
Another competitor for landing astronauts on the Moon has been Blue Origin. While they have demonstrated suborbital flights, with vertical landings, they have not yet demonstrated heavy lift capability. Their New Glenn booster has had many delays and now seems to be scheduled for a first launch late in 2023.
Blue Origin has developed completely new engines, the BE-4 for the first stage which will be powered by seven of them. In October of 2022 Blue Origin delivered two of these engines to a customer, ULA, for the new generation Vulcan Centaur rocket. It is expected to launch with them early in 2023. This rocket has solid boosters as well as the BE-4 engine so uses less BE-4’s than New Glenn.
In any case, the delivery of these engines to a paying customer seems like a positive development for the eventual flight of New Glenn.
Generic Predictions (Jan 01, 2023)
Here are some new predictions, not really as detailed as the ones from 2018, but they are answers to much of the hype that is out there. I have not given time lines as these are largely “not going to happen the way the zeitgeist suggests”.
- The metaverse ain’t going anywhere, despite the tens of billions of dollars poured in. If anything like the metaverse succeeds it will from a new small player, a small team, that is not yoked down by an existing behemoth.
- Crypto, as in all the currencies out there now, are going to fade away and lose their remaining value. Crypto may rise again but it needs a new set of algorithms and capability for scaling. The most likely path is that existing national currencies will morph into crypto currency as contactless payment become common in more and more countries. It may lead to one of the existing national currencies becoming much more accessible world wide.
- No car company is going to produce a humanoid robot that will change manufacturing at all. Dexterity is a long way off, and innovations in manufacturing will take very different functional and process forms, perhaps hardly seeming at all like a robot from popular imagination.
- Large language models may find a niche, but they are not the foundation for generally intelligent systems. Their novelty will wear off as people try to build real scalable systems with them and find it very difficult to deliver on the hype.
- There will be human drivers on our roads for decades to come.
And One Last BITE
I know, people are disappointed that I didn’t say anything in this post about ChatGPT more substantial than “calm down”. But really I don’t think there is a lot worth saying. People are making the same mistake that they have made again and again and again, completely misjudging some new AI demo as the sign that everything in the world has changed.
It hasn’t.
In essence ChatGPT is picking the most likely word to follow all those that precede it, whether it is the words in a human input prompt, or what it has already generated itself. The best analogy that I see for it is that ChatGPT is a dream generator, the dreams that you have at night when you are asleep.
Sometimes from your dreams you can pick out an insight about yourself or the world around you. Or sometimes your dreams make no sense at all, and sometimes they can be scary nightmares. Basing your life, unquestioned, on what you dreamed last night, and every night, is not a winning strategy.