[An essay in my series on the Future of Robotics and Artificial Intelligence.]
Much of the recent enthusiasm about Artificial Intelligence is based on the spectacular recent successes of machine learning, itself often capitalized as Machine Learning, and often referred to as ML. It has become common in the technology world that the presence of ML in a company, in a development process, or in a product is viewed as a certification of technical superiority, something that will outstrip all competition.
Machine Learning is what has enabled the new assistants in our houses such as the Amazon Echo (Alexa) and Google Home by allowing them to reliably understand as we speak to them. Machine Learning is how Google chooses what advertisements to place, how it saves enormous amounts of electricity at its data centers, and how it labels images so that we can search for them with key words. Machine learning is how DeepMind (a Google company) was able to build a program called Alpha Go which beat the world Go champion. Machine Learning is how Amazon knows what recommendations to make to you whenever you are at its web site. Machine Learning is how PayPal detects fraudulent transactions. Machine Learning is how Facebook is able to translate between languages. And the list goes on!
While ML has started to have an impact on many aspects of our life, and will more and more so over the coming decades, some sobriety is not out of place. Machine Learning1 is not magic. Neither AI programs, nor robots, wander around in the world ready to learn about whatever there is around them.
Every successful application of ML is hard won by researchers or engineers carefully analyzing the problem that is at hand. They select one or many different ML algorithms, and custom design how to connect them together and to the data. In some cases there is an extensive period of training on very large sets of data before the algorithm can be run on the problem that is being solved. In that case there may be months of work to do in collecting the right sort of data from which ML will actually learn. In other cases the learning algorithm will be integrated in to the application and will learn while doing the task that is desired–it might require some training wheels in the early stages, and they too must be designed. In any case there is always a big design project about how, when the ultimate system is operational, the data that comes in will be organized, processed and mapped before it reaches the ML component of the system.
When we are tending plants we pour water on them and perhaps give them some fertilizer and they grow. I think many people in the press, in management, and in the non-technical world have been dazzled by the success of Machine Learning, and have come to think of it a little like water or fertilizer for hard problems. They often mistakenly believe that a generic version will work on any and all problems. But while ML can sometimes have miraculous results it needs to be carefully customized after the DNA of the problem has beed analyzed. And even then it might not be what is needed–to extend the metaphor, perhaps it is the climate that needs to be adjusted and no amount of fertilizer or ML will do the job.
How does Machine Learning work, and is it the same as when a child or adult learns something new? The examples above certainly seem to cover some of the same sort of territory, learning how to understand a human speaking, learning how to play a game, learning to name objects based on their appearance.
Machine Learning started with games
In the early 1940’s as war was being waged world wide there were only a handful of electronic digital computers in existence. They had been built, using the technology of vacuum tubes, to calculate gunnery tables and to decrypt coded military communications of the enemy. Even then, however, people were starting to think about how these computers might be used to carry out intelligent activities, fifteen years before the term Artificial Intelligence was first floated by John McCarthy.
Alan Turing, who in 1936 had written the seminal paper that established the foundations of modern computation, and Donald Michie, a classics student from Oxford (later he would earn a doctorate in genetics), worked together at Bletchley Park, the famous UK code breaking establishment that Churchill credited with subtracting years from the war. Turing contributed to the design of the Colossus computer there, and through a key programming breakthrough that Michie made, the design of the second version of the Colossus was changed to accommodate his ideas even better. Meanwhile at the local pub the pair had a weekly chess game together and discussed how to program a computer to play chess, but they were only able to get as far as simulations with pen and paper.
In the United States right after the war, Arthur Samuel3, an expert on vacuum tubes was the leader of an effort to built the ILLIAC computer at the University of Illinois at Urbana-Champaign. While the computer was still being built he planned out how to program it to play checkers (or draughts in British English), but left in 1949 to join IBM before the University computer was completed. At IBM he worked on both vacuum tubes and transistors to bring IBM’s first commercial general purpose digital computers to market. On the side he was able to implement a program that by 1952 could play checkers against a human opponent. This was one of the first non-arithmetical programs to run on general purpose digital computers, and has been called the first AI program to run in the United States.
Samuel continued to improve the program over time and in 1956 it was first demonstrated to the public. But Samuel wondered whether the improvements he was making to the program by hand could be made by the machine itself. In 1959 he published a paper titled “Some Studies in Machine Learning Using the Game of Checkers”2, the first time the phrase “Machine Learning” was used–earlier there had been models of learning machines, but this was a more general concept.
The first sentence in his paper was: “The studies reported here have been concerned with programming of a digital computer to behave in a way which, if done by human beings or animals, would be described as involving the process of learning.” Right there is his justification for using the term learning, and while I would not quibble with it, I think that it may have had some unintended consequences which we will explore towards the end of this post.
What Samuel had realized, demonstrated, and exploited, was that digital computers were by 1959 fast enough to take over some of the fine tuning that a person might do for a program, as he had been doing since the first version of his program in 1952, and ultimately eliminate the need for much of that effort by human programmers by letting the computer do some Machine Learning on appropriate parts of the problem. This is exactly what has lead, almost 60 years later to the great influence that ML is now having on the world.
One of the two learning techniques Samuel described was something he called rote learning, and today would be labelled as a well known programming technique called memoization4, and sped up the program. The other learning technique that he investigated involved adjusting numerical weights on how much the program should believe each of over thirty measures of how good or bad a particular board position was for the program or its human opponent. This is closer in spirit to techniques in modern ML. By improving this measure the program could get better and better at playing. By 1961 his program had beat the Connecticut state checker champion. Another first for AI, and enabled by the first ML program.
Arthur Samuel built his AI and ML systems not as an academic researcher but as a scholar working on his own time apart from his day job. However he had an incredible advantage over all the AI academic researchers. Whereas access to computers was rare and precious for them, Samuel’s day job was as a key participant building the first mass produced digital computers, and each one needed to be run for many hours to catch early life defects before it could be shipped. He had a surfeit of free computer time. Just about no one else in the world had such a luxurious computational environment.
Sometimes the less lucky academics had to resort to desperate measures. And so it was for Donald Michie, colleague of Alan Turing back at Bletchley Park. By 1960 he was a Senior Lecturer in Surgical Science at the University of Edinburgh, but his real interests lay in Artificial Intelligence, though he always preferred the term Machine Intelligence.
In 1960 Surgical Science did not have much pull in getting access to a digital computer. So Donald Michie himself built a machine that could learn to play the game of tic-tac-toe (Noughts and Crosses in British English) from 304 matchboxes, small rectangular boxes which were the containers for matches, and which had an outer cover and a sliding inner box to hold the matches. He put a label on one end of each of these sliding boxes, and carefully filled them with precise numbers of colored beads. With the help of a human operator, mindlessly following some simple rules, he had a machine that could not only play tic-tac-toe but could learn to get better at it.
He called his machine MENACE, for Matchbox Educable Noughts And Crosses Engine, and published5 a report on it in 1961. In 1962 Martin Gardner6 reported on it in his regular Mathematical Games column in Scientific American, but illustrated it with a slightly simpler version to play hexapawn, three chess pawns against three chess pawns on a three by three chessboard. This was a way to explain Machine Learning and provide an experimental vehicle to the scientifically interested lay population, who certainly would not have had access to a digital computer at that time. Gardner suggested that people try building a matchbox computer to play simplified checkers with two pieces for each player on a four by four board. But he felt that even the simplest version of chess that he could come up with, on a five by five board would require too many matchboxes to be practical.
I first read about the matchbox computer in 1967 in a book7 published the previous year which was written by a group of teachers at a British high school. They neither attributed the idea to Michie, nor the game they described it learning, hexapawn, to Gardner. As a barely teenager who had to hand build every machine for every experiment I wanted to do in AI, I must admit I thought that the matchbox computer was too simple a project and so did not pursue it. Now, however, I have come to realize that it is the perfect way of introducing how Machine Learning works, as everything is there to see. Even though MENACE is over fifty years old many of the problems that it faced are still relevant to machine learning algorithms today, and it shares many characteristics with almost all of today’s machine learning. Due to its simplicity it can be described in complete detail and no mathematics is needed to get a strong intuitive understanding of how it works.
Today people generally recognize three different classes of Machine Learning, supervised, unsupervised, and reinforcement learning, all three very actively researched, and all being used for real applications. Donald Michie’s MENACE introduced the idea of ML reinforcement learning, and he explicitly refers to reinforcement as a key concept in how it works.
How a collection of matchboxes plays & learns
I am going to take the details I give here from a retelling of how MENACE worked from a more accessible 1963 paper8. In a very often republished picture from that paper Donald Michie (or at least his hands) can be seen both playing tic-tac-toe against the machine, and operating the machine.
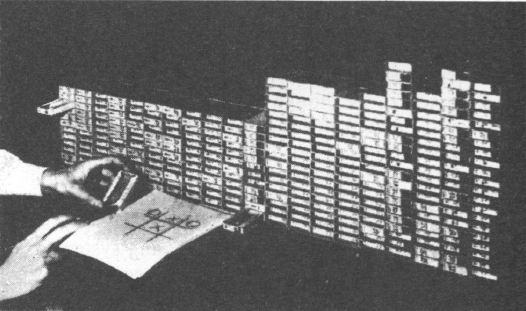
On the sheet of paper in from of him you can just see a large tic-tac-toe diagram. There are stacks of matchboxes toward the rear of the table, must likely glued together so that they stay in place. Some of the matchboxes have their drawers partially pulled out, and he is holding one of the drawers in his left hand. As we will see this image captures most of what is going on as MENACE learns to play better and better tic-tac-toe.
To make the description more clear I am going to introduce a second person; let’s call him Alan. Alan will operate the matchbox machine according to fixed rules, and will not have to make any decisions that are not determined completely by those rules. Donald, the human player will not touch the machine, but instead will write out the moves on a standard three by three grid, accepting the moves of MENACE as delivered to him by Alan, playing his own moves, and being the adjudicator of when he or MENACE has won by getting three in a row.
Michie had MENACE always play first, with a ‘O’ and so we will do that here also. Below is what a game might look like, starting with an empty board, MENACE playing first in the middle of the top row, Donald replying, with an ‘X’ in the top right corner as his first play to be made, and back and forth. Notice that I am using a period, or ‘.’, for a blank, so that I don’t have to draw the customary horizontal and vertical lines to divide the squares. I have put a little indicator under each board position where it is MENACE’s turn to play. At MENACE’s third move it blocks an immediate win where Donald would be able to complete the diagonal from the upper right to the lower left, but Donald replies with a move to the bottom right corner, threatening now two possible three in a rows on his next turn, and MENACE is able to block only one of them so MENACE loses to Donald.
... .O. .OX .OX .OX .OX .OX OOX OOX
... ... ... ... .X. .X. .X. .X. .XX
... ... ... .O. .O. OO. OOX OOX OOX
^ ^ ^ ^
The way that MENACE plays is that there is a matchbox for every possible board configuration that could arise in the course of the game when it is MENACE’s turn. There is a box for every configuration where it is MENACE’s first, second, third, or fourth turn, but not for its fifth turn as it has no choice to make there as there will only be one empty square.
The configurations are drawn on a small label pasted to the front of each individual drawer. When it is MENACE’s turn, Alan finds the matchbox with a label that matches the current state of play on the piece of paper on which Donald is keeping track of the game. He opens the drawer which has some number of colored beads in it. Without looking he randomly picks one of the beads. Importantly he leaves the drawer open and after showing the bead to Donald he puts it on the table in front of the open drawer from which it came. The boxes are arranged left to right corresponding to less moves played and then more moves played so it is easy to keep tack of which bead came from which drawer. There are nine colors of bead, and each color corresponds to one of the nine squares in tic-tac-toe. After seeing the bead Donald writes down an ‘O’ at the appropriate square on his piece of paper, and then writes his own ‘X’ as his next move, and the cycle repeats.
Although the actual colors of the beads do not really matter, here are the colors and their correspondence to the squares that were used in the original experiments (this time I have put in the horizontal vertical lines to divide the color words).
white | lilac | silver
-----------------------
black | gold | green
-----------------------
amber | red | pink
How many beads are there in each box? For all the first move boxes, of which there is one, corresponding to the empty board, there are four beads for each possible move, so 36 in total. For all possible second moves for MENACE there are only seven possibilities, and each of those empty squares has three beads. For the third move there are 2 beads for each of the five possibilities, and for each fourth move there is one beed for each of the three possibilities.
To start out MENACE is playing each move completely at random. But suppose MENACE loses a game. Then Alan discards the beads below each open drawer and closes them all. This is negative reinforcement as MENACE lost, and so made moves it should not make in the future. In particular its fourth move, with only one bead, led to a loss that was at that point completely out of its control. So removing that bead means that MENACE will never play that bad move again. Its third move was perhaps a little suspect so that goes down to only one bead instead of two and it is less likely to try that again, but if it does it will not be tricked in exactly the same way again.
If MENACE draws the game then it gets positive reinforcement as each bead that was picked from each drawer is put back in, along with an extra bonus bead of the same color. If it won the game then it gets three additional beads same colored beads along with the one played at each turn. In this way MENACE tends to do the things that worked in the past, but if the opponent (in this case Donald) finds a new way to win against what used to work then MENACE will gradually adapt to that and avoid that losing line of play.
That is it. With Alan following this simple set of rules MENACE learns to play better and better over time. But there is one point of practicality.
Human structuring of the learning problem
As I described it above there are a lot of matchboxes needed for MENACE. There is 1 for MENACE’s first move, 72 for its second move, 756 for its third, and 1372 for its fourth move, for a total of 2201 matchboxes.
But let’s look at another possible game, where every position is different from the previous game we looked at.
... ... ... ... ... O.. O.. O.O O.O
... ..O ..O O.O OXO OXO OXO OXO OXO
... ... ..X ..X ..X ..X X.X X.X XXX
^ ^ ^ ^
But wait, this is really the same game as before just rotated ninety degrees clockwise. It is going to take a lot longer to learn how to play tic-tac-toe if MENACE has to independently learn that its second move in this game is just as bad as its second move in the previous game. To make MENACE learn faster, and to reduce the number of matchboxes down to a more manageable level, Donald Michie took into account that up to eight different patterns of Noughts and Crosses might really be essentially the same.
Here is an example where an original board positions is rotated clockwise by a quarter, half, and three-quarter turn, and where a reflection about the vertical axis, the horizontal axis, and the two diagonal axes all give different board positions Nonetheless these eight positions are essentially the same as far as the rules of the game of tic-tac-toe are concerned.
OX. ..O ... ... .XO ... O.. ...
.O. .OX .O. XO. .O. .O. XO. .OX
... ... .XO O.. ... OX. ... ..O
Some board positions may not result in so many different looking positions when rotated or reflected. For instance, a single play in the center of the board is not changed at all by these spatial transformations.
In any case, by considering all the rotations and reflections of a board position as a single position, and therefore only assigning one matchbox to all of them combined, the requirements for MENACE are reduced to 1 matchbox, as before, for MENACE’s first move, 12 for its second, 108 for its third, and 183 for its fourth move, bringing the total9 to 304. Furthermore by looking at the symmetries in what move is played there are often less essentially different moves than there are empty squares. For instance in both these cases MENACE is about to play an ‘O’:
... .O.
... .O.
... X.X
In each case there are only three essentially different moves that can be played, so MENACE’s matchboxes for these move need only start out with three different colored beads rather than nine or five respectively.
By taking into account these symmetries the MENACE machine can be much smaller, and the speed of learning will be much faster, as lessons from one symmetric position will be automatically learned at another. The cost is that Alan is going to have to do quite a bit more work to operate MENACE. Now he will have to look at the position that Donald shows on the piece of paper where Donald is playing and not just look for an identical label on the front of a matchbox, but look for one that might be a rotation or reflection of the state of the game. Then, when Alan randomly selects a bead which indicates a particular move on the label on the matchbox from which it game, he will have to figure out which square that corresponds to on Donald’s sheet of paper through the inverse rotation or reflection that he used to select the matchbox. Fortunately this extra work is all quite deterministic and Alan is still following a strict set of rules with no room for judgement to creep in. We will come back to this a little later and mechanize Alan’s tasks though a few sheets of very simple instructions that will do all this extra work.
How well do matchboxes learn?
MENACE is learning what move it should choose in one of 304 essentially different board configurations for its first four moves in a game of tic-tac-toe. Since Alan randomly picks out one bead from the matchbox corresponding to one of those configurations it is making a random move from a small number of moves but the probability of a particular move goes up when there are more beads of a particular color from positive reinforcements from previous games, and the probability of a move which leads to a loss goes down relative to the other possible moves as its beads are removed.
Look back at the two examples just above for a first move and a third move for MENACE. The empty board starts out with 12 beads, for each of three different colors representing placing an ‘O’ on a corner, in the middle of an edge, or in the middle of the board. The board waiting for MENACE’s third ‘O’ to be played starts out with just six beads, of three different colors, corresponding to playing the blocking move between the ‘X’s, one of the corners, or one of the other two middle edges. We will refer to number of beads of the same color in a single box as a parameter. By mapping all symmetric situations to a common matchbox and restricting the different moves to essentially different moves, there are 1087 parameters that MENACE adjusts over time through the removal or addition of beads. When MENACE starts off it has a total of 1720 beads representing those 1087 parameters in 304 different matchboxes.
When MENACE starts out it is playing uniformly randomly over all essentially different moves. If two uniformly random players play against each other, the first to play wins 59% of the time, draws 13%, and loses 28%. This shows the inherent bias in the game for the first player, which makes learning a little easier for MENACE.
In his original paper Michie reported that MENACE became quite a good player after only 220 games and was winning most games, but neither I nor others who report simulating MENACE (you can find many with a web search) saw MENACE doing that well at all. In fact since a perfect player never loses at tic-tac-toe then two perfect players always draw the game 100% of the time. It seems likely that Michie was carefully training MENACE with deliberately chosen games, and then playing against it in a fairly random way. He alludes to this when he later converted to a computer simulation of MENACE and mentions that playing against random moves results in much slower learning than playing against a deliberate policy.
To explore this I made a computer simulation of MENACE and three different simulated strategies of Donald playing against MENACE. I let learning proceed for 4,000 games, and did this multiple times against each of the three simulated players. Since there is randomness in picking a bead from a matchbox, the random number generator used by the computer to simulate this ensures that different trials of 4,000 games will lead to different actual games being played. Every so often I turned off the learning and tested how well10 MENACE was currently playing against the three simulated players, including the two it was currently not learning from.
The three simulated players were as follows. Player A played completely randomly at all times. Player B played optimally and was unbeatable. Player C was optimal except that 25% of its moves were random instead of the optimal play. These are the three different versions of Donald that I used in my simulations.
In the table below the first row shows the performance of MENACE before it has learned at all, against each of the three simulated players. Each triple of numbers is the percentage of wins, draws, and losses (these are rounded percentages from a very large number of test games so don’t necessarily add to exactly 100%). As expected it never wins against Player B which plays optimally and can not be beaten. Player C which makes mistakes 25% of the time can be beaten, but only about a quarter of the time. In each row below that, MENACE was trained from scratch playing 4,000 games against a different one of these players. In each column we show, with MENACE stopped from further learning and adjusting its parameters, how it typically did against each of the three players once trained. We say “typically” as there is some variation in the resulting percentages between different trials with the same conditions, but only by a few points, and not in all cases.
\ compete | | | |
\ against | Player A | Player B | Player C |
\------\ | | | |
trained \ | | | |
against \| | | |
------------|-------------|-------------|-------------|
no training | 59/ 13/ 28 | 0/ 24/ 76 | 27/ 19/ 53 |
============|=============|=============|=============|
Player A | 86/ 8/ 6 | 0/ 28/ 72 | 50/ 20/ 30 |
------------|-------------|-------------|-------------|
Player B | 71/ 15/ 14 | 0/100/ 0 | 38/ 48/ 14 |
------------|-------------|-------------|-------------|
Player C | 90/ 8/ 2 | 0/ 99/ 1 | 56/ 42/ 2 |
------------|-------------|-------------|-------------|
The first thing to notice is that how MENACE plays really does depend on what player it was trained against. When it is trained against Player B, which always plays optimally, it very quickly, usually after only about 200 games, learns to always play to a draw. But with that training (look in the same row) it is really not very good at playing against Player C which plays optimally with a 25% error rate. That is probably because in its training it never got to win at all against Player B, so it has not learned any winning moves to use against Player C.
When MENACE is trained against Player A (look in the row labelled Player A), which plays completely randomly it does learn to play against it quite well, and it also does reasonably well against Player C, probably because it has accidentally won enough times during training to have boosted some winning moves when they are available. It does dismally against the optimal Player B however. This particular box in the table has the highest variance of all in the table. Sometimes after 4,000 games it is doing less than half as well against Player B than when it started out learning.
When MENACE trains against Player C it does the best overall. It sees enough losses early on that after about 400 games it is starting to get good at avoiding losses, though it is still slowly, slowly getting better at that aspect of its game even after 4,000 games of learning. It usually doesn’t get quite as good as Player B, and very occasionally still loses to it, but it is really good at winning when there are opportunities for it to do so. We can see that against Player C it as learned to take advantage of its mistakes to drive home a win.
While not as good as a person, MENACE does get better against different types of players. It does however end up tuning its game to the type of player it is playing against.
There is also something surprising about the number of beads. MENACE starts off with 1,720 beads, but depending on which of Players A, B, or C, it is learning from it has from 2,300 to 3,200 beads after just 200 games, and always there is at least one parameter with over one hundred beads representing it by that time. By 4,000 games it may have more than 35,000 beads representing just 1,087 parameters, with as many as 6,500 beads for one of the parameters. This seems unnecessary, and perhaps the impact of rewarding all the moves with three beads on a win. However when I changed my simulation to never add more beads to a parameter that already had at least one hundred beads, a practical limit perhaps for a MENACE machine built from physical matchboxes, it tended to slow down learning in most cases represented in the table above, and even had small drops in typical levels of play even after 4,000 games of experience when playing against Players A and C.
Note that besides eliminating ever taking the very last bead away from a matchbox after a loss, this is the only place where I deviated from Michie’s description of his MENACE. Since he chose his plays carefully to instruct MENACE, and since he only played 220 games by hand, he perhaps did not come across the phenomenon of large numbers of beads.
Mechanizing MENACE a little more
In preparation for comparing how MENACE learns to how a person learns I want to make the role of Alan, the human operator of MENACE, a little clearer. In the description derived from Michie’s original paper, Michie himself played the role of both Donald and Alan. In my description above I talked about Alan matching the image of the paper on which Donald was playing to the labels on the matchboxes, possibly having to rotate or reflect the game board. And after randomly selecting a bead from that box, Alan would need to figure out which square that applied to on Donald’s piece of paper.
That sounds a little fuzzy, and perhaps requiring some reasoning on Alan’s part, so now we’ll make explicit a very rule driven approach that we could enforce, to ensure that Alan’s role is completely rote, with no judgement at all required.
We will make the communication between Donald and Alan very simple. Donald will hand Alan a string of nine characters drawn from ‘.’, ‘O’, and ‘X’, representing the board position after his play, and Alan will hand back a string where one of the periods has been replaced by an ‘O’. To enable this we will number the nine positions on the tic-tac-toe board as follows.
123
456
789
The string representing the board is just the contents of these squares in numerical order. So, for instance, if Donald has just played his ‘X’ to make the following board position, then he should give Alan the string printed to the right.
...
.OX ....OX...
...
We will get rid of the labels, images of tic-tac-toe board positions from the front of the match boxes, and replace them with the numbers 1 through 304, so that each matchbox has a unique numerical label. We will label the matchbox corresponding to the empty board with 1, as that will be how Alan starts a game, by drawing a bead from there, and he will look up what square that color means in a “Transform #1” on a sheet of paper with eight different transforms listed. Here they are:
Transform #1:
white =1 lilac =2 silver=3
black =4 gold =5 green =6
amber =7 red =8 pink =9
Transform #2:
white =3 lilac =6 silver=9
black =2 gold =5 green =8
amber =1 red =4 pink =7
Transform #3:
white =9 lilac =8 silver=7
black =6 gold =5 green =4
amber =3 red =2 pink =1
Transform #4:
white =7 lilac =4 silver=1
black =8 gold =5 green =2
amber =9 red =6 pink =3
Transform #5:
white =3 lilac =2 silver=1
black =6 gold =5 green =4
amber =9 red =8 pink =7
Transform #6:
white =7 lilac =8 silver=9
black =4 gold =5 green =6
amber =1 red =2 pink =3
Transform #7:
white =1 lilac =4 silver=7
black =2 gold =5 green =8
amber =3 red =6 pink =9
Transform #8:
white =9 lilac =6 silver=3
black =8 gold =5 green =2
amber =7 red =4 pink =1
The eight transforms correspond to four rotations (of zero, one, two and three quarters clockwise), and four reflections.
The remaining 303 matchboxes correspond to the essentially different board positions for MENACE’s second, third, and fourth moves. Although there are 72 different board positions for MENACEs second move there are only twelve that essentially distinct, and here they all are, numbered 2 through 13 as the next twelve matchboxes after the one for the first move.
#1 #2 #3 #4 #5 #6 #7 #8 #9 #10 #11 #12 #13
| | | | | | | | | | | | |
... .O. .O. .O. .O. X.. .X. OX. O.X O.. O.. O.. XO.
... X.. .X. ... ... .O. .O. ... ... .X. ..X ... ...
... ... ... X.. .X. ... ... ... ... ... ... ..X ...
When Alan is given a string by Donald (there is only one possible string for the first move, the empty board, but there are 72 possibilities for the MENACE’s second move, 756 for the third, and 1372 for the fourth move) Alan just mindlessly looks it up in a big table that is printed on a few sheets of paper. Each line has a string representing a board position, a box number, and a transform number. For instance, for the second move for MENACE we talked about above with string ....OX...
Alan would find it, simply by matching character for character, in the following part of the table (for the first and second moves by MENACE):
......... Box: # 1, Transform #1
.......OX Box: # 13, Transform #3
.......XO Box: # 8, Transform #3
......O.X Box: # 9, Transform #6
......OX. Box: # 8, Transform #6
......X.O Box: # 9, Transform #3
......XO. Box: # 13, Transform #6
.....O..X Box: # 13, Transform #8
.....O.X. Box: # 2, Transform #8
.....OX.. Box: # 4, Transform #8
.....X..O Box: # 8, Transform #8
.....X.O. Box: # 2, Transform #3
.....XO.. Box: # 11, Transform #6
....O...X Box: # 6, Transform #3
....O..X. Box: # 7, Transform #3
....O.X.. Box: # 6, Transform #4
....OX... Box: # 7, Transform #2 <== this one
....X...O Box: # 10, Transform #3
....X..O. Box: # 3, Transform #3
....X.O.. Box: # 10, Transform #4
....XO... Box: # 3, Transform #2
...O....X Box: # 4, Transform #4
...O...X. Box: # 2, Transform #4
...O..X.. Box: # 13, Transform #4
...O.X... Box: # 5, Transform #4
...OX.... Box: # 3, Transform #4
...X....O Box: # 11, Transform #3
...X...O. Box: # 2, Transform #6
...X..O.. Box: # 8, Transform #4
...X.O... Box: # 5, Transform #2
...XO.... Box: # 7, Transform #4
..O.....X Box: # 9, Transform #2
..O....X. Box: # 11, Transform #2
..O...X.. Box: # 12, Transform #2
..O..X... Box: # 8, Transform #2
..O.X.... Box: # 10, Transform #2
..OX..... Box: # 11, Transform #5
..X.....O Box: # 9, Transform #8
..X....O. Box: # 4, Transform #3
..X...O.. Box: # 12, Transform #4
..X..O... Box: # 13, Transform #2
..X.O.... Box: # 6, Transform #2
..XO..... Box: # 4, Transform #7
.O......X Box: # 4, Transform #5
.O.....X. Box: # 5, Transform #1
.O....X.. Box: # 4, Transform #1
.O...X... Box: # 2, Transform #5
.O..X.... Box: # 3, Transform #1
.O.X..... Box: # 2, Transform #1
.OX...... Box: # 13, Transform #5
.X......O Box: # 11, Transform #8
.X.....O. Box: # 5, Transform #3
.X....O.. Box: # 11, Transform #4
.X...O... Box: # 2, Transform #2
.X..O.... Box: # 7, Transform #1
.X.O..... Box: # 2, Transform #7
.XO...... Box: # 8, Transform #5
O.......X Box: # 12, Transform #1
O......X. Box: # 11, Transform #7
O.....X.. Box: # 9, Transform #7
O....X... Box: # 11, Transform #1
O...X.... Box: # 10, Transform #1
O..X..... Box: # 8, Transform #7
O.X...... Box: # 9, Transform #1
OX....... Box: # 8, Transform #1
X.......O Box: # 12, Transform #3
X......O. Box: # 4, Transform #6
X.....O.. Box: # 9, Transform #4
X....O... Box: # 4, Transform #2
X...O.... Box: # 6, Transform #1
X..O..... Box: # 13, Transform #7
X.O...... Box: # 9, Transform #5
XO....... Box: # 13, Transform #1
This tells Alan that the move given to him by Donald is to be played by matchbox #7, and then he is to use Transform #2, which we saw above, to interpret the color of the drawn move as to which square is meant. We can see what position box #7 corresponds to above, though Alan does not know that. He simply reaches into box #7 and pulls out a random bead. As it happens, in my simulation of MENACE where it never tries to play two essentially the same moves, the only beads in #7 are colored white, black, amber, and red, corresponding to essentially different moves down the left column and in the bottom at the middle using the original MENACE bead color interpretations. Under Transform #2 we see that those colors correspond to squares 3, 2, 1, and 4, respectively, which are across the top row and the left middle square for the way Donald is playing. So whichever one of those colors is removed from the box, Alan simply goes to that position in the string that was given to him by Donald, and changes the blank to an ‘O’. So suppose that Alan pulls out a black bead. In that case he changes the second element to an ‘O’, and gives it back to Donald who then interprets the string to mean the following new board position:
.O.
.O..OX... .OX
...
The only remaining thing is the reinforcement signal. Donald, the human player, is the one who is responsible for deciding when the game is over and at that point needs to communicate one of just three options to Alan; L, for loss, meaning forfeit all the beads out of boxes, D, for draw, meaning put the beads back with an extra one of the same color for each, or W, for win, meaning put them back with three extra ones of each.
Summary of What Alan Must Do
With these modifications we have made the job of Alan both incredibly simple and incredibly regimented.
-
- When Donald gives Alan a string of nine characters Alan looks it up in a table, noting the matchbox number and transform number.
- He opens the numbered matchbox, randomly picks a bead from it and leaves it on the table in front of the open matchbox.
- He looks up the color of the bead in the numbered transform, to get a number between one and nine.
- He replaces that numbered character in the string with an ‘O’, and hands the string back to Donald.
- When Donald gives Alan a sign for one of L, D, or W, Alan does the following:
- For L he removes the beads on the table and closes the open matchboxes.
- For D he adds one more bead of the same color to each one on the table, and puts the pairs in the matchboxes behind them, and closes the matchboxes.
- For W he adds three more beads of the same color to each one on the table, and puts the sets of four in the matchboxes behind them, and closes the matchboxes.
That is all there is. Alan looks up things on a few sheets of paper, acts on matchboxes, and changes a character in a string.
One could say that Alan is a Turing machine.
The thing that learns how to play tic-tac-toe is a combination of Alan following these completely strict rules, and the contents of the matchboxes, the colored beads, whose number varies over time.
Is this how a person would learn?
For anyone who has played tic-tac-toe the most striking thing about about the way that MENACE learns is that it has no concept of “three in a row”. When we teach a child how to play the game that is the most important thing to explain, showing how rows, columns, and diagonals can all give rise to three O’s or X’s in a row. We explain to the child that getting three in a row is the goal of the game. So the first rule for playing tic-tac-toe is to complete three in a row on your move if that option is available. MENACE does not know this at all.
The next thing, or second rule, we might show our tic-tac-toe pupil is that assuming they have no winning move, the next best thing is to block the opponent if they have two of three in a row already with an empty spot to play and complete it. This does not guarantee an eventual win, as there are seventeen essentially different situations where the ‘X’ player may have two three-in-a-row’s ready to play, and the ‘O’ player can only block one of them. Here are two examples of that.
.O. XOO
OOX O..
.XX .XX
However just these two rules are a marked improvement over random play. If we play tic-tac-toe with the preference of rule 1 if it is applicable, then rule 2 if that is applicable, and if neither is applicable then make a random move, we actually get a pretty good player. Here is the same sort of table as above, with an identical first row showing how well random untrained play succeeds against Players A, B, and C, then in the second row how well the addition of rules 1 and 2 improve a random player
| Player A | Player B | Player C |
------------|-------------|-------------|-------------|
random play | 59/ 13/ 28 | 0/ 24/ 76 | 27/ 19/ 53 |
============|=============|=============|=============|
+ rules 1&2 | 86/ 10/ 4 | 0/ 82/ 18 | 51/ 37/ 13 |
------------|-------------|-------------|-------------|
Just the addition of those two rules gets to a level of play against a random player (Player A) that MENACE only gets to after about 4,000 games learning from Player A. Against Player B, the optimal player, it does not get as good as it does when it is trained for 200 games by Player B, but it is better against either of the other two players than when it has been been trained for 4,000 games against Player B. And against Player C, the player with 25% error rate from optimal play, it is almost as good as it ever gets even being trained by Player C.
Clearly these rules are very powerful. But they are also rather easy for a child to learn as they don’t require thinking ahead beyond the very next move. All the information is right there in the board layout, and there is no need to think ahead about what the opponent might do next once the current move is made. What is it that the child has that MENACE does not?
One answer might be geometrical representations. MENACE does not have any way to represent “three in a row” as a concept that can be applied to different situations. Each matchbox is a kingdom unto itself about one particular essentially unique board configuration. If one particular matchbox learns, through reinforcement, that it is good to place a third ‘O’ to make a diagonal, there is no way to transfer that insight, were MENACE able to have it, to other essentially different situations where there is also a diagonal that can be filled in. And certainly not to a situation about completing a horizontal or vertical row.
As we saw, Michie did incorporate some geometric “knowledge” into MENACE by mapping all rotations and reflections of the tic-tac-toe board to a common matchbox. But the machine itself has no insight into this–it was all done ahead of time by Michie (whose preparation was extended slightly by me so that Alan could be very explicitly machine-like in his tasks) by producing the dictionary of positions that mapped to matchboxes number 1 through 304, and which of the eight inversion lookup tables that mapped from color of bead to numbered square on the board should be used. That manual design process handled some mappings between different aspects of three in a row but not all. In general a researcher or engineer using Machine Learning to solve a problem does something very similar, in reducing the space of inputs. The art of it is to reduce the input space so that learning can happen more quickly, but not over reduce the space so that subtle differences in situations are obliterated by the pre-processing. By mapping from all the general board positions to precisely those that are essentially different, Donald Michie, the Machine Learning engineer in this case, managed so satisfy both those goals.
A child knows something about geometry in a way that MENACE does not. A child can talk about things being in a row independently of learning tic-tac-toe. A child has learned that in-a-row-ness is independent of orientation of the line the defines the row. By a certain age a child comes to know that the left-to-rightness of some ordering depends on the point of view of the observer, so they are able to see that two in a row with an empty third one is an important generalization that applies equally to the horizontal and vertical rows around the edges, thinking about them in both directions, and also applies to the horizontal and vertical rows that go through the middle square, and to the two diagonals that also go through that square. The child may or may not generalize that to two at each end of a row with the middle to be filled in–perhaps that might be a different concept for young children. But the rowness of things is something they have a lot of experience with, and are able to apply to tic-tac-toe. In computer science we would talk about rowness being a first class object for a child–something that can be manipulated by other programs, or in a child by many cognitive systems. In MENACE rowness is hidden in the pre-analysis of the problem that Donald Michie did in order to map tic-tac-toe to collection of numbered matchboxes with beads in them.
The learning that MENACE does somehow feels different to the learning that human does when playing tic-tac-toe. That is not to say that all learning that a human does is necessarily completely different from what MENACE does. Perhaps things that humans learn in an unconscious fashion (e.g., how to adjust their stance to stay balanced–negative and positive reinforcement signals based on whether they hit the ground or not), where we have no way to access what is happening inside us, nor an ability to talk about it, is more like MENACE learning.
Not all learning is necessarily the same sort of learning.
Is this how a person would play?
A more fundamental question, perhaps, is whether MENACE plays tic-tac-toe like a person does, and I think the answer is a clear no. The MENACE system consisting of the matchboxes and Alan strictly following rules only fills in part of the role of a normal player. The rest of what is usually a social interaction between two people is all taken on by Donald.
There is no representation inside the MENACE (where we include in the definition of MENACE the sheets of papers that Alan consults, and the rules that we have instructed Alan to strictly follow) of tic-tac-toe being a game that is played. MENACE does not know what a game is, or even that it is playing a game. All that happens inside MENACE is that one at a time, either three or four times sequentially, one of its matchbox drawers is opened and a bead is randomly removed, and then either the beads are taken away, or they are put back in the boxes from where they came with either one or three additional beads of the same color, and the boxes are closed.
All the gameness of tic-tac-toe is handled by the human Donald. It is he who initiates the game by handing Alan a string of nine periods. It is he who manages the consistency of subsequent turns by annotating his hand drawn tic-tac-toe board with the moves. It is he who decides when the game has been won, drawn, or lost, and communicates to Alan the reinforcement signal that is to be applied to the open matchboxes. It is he, Donald, who decides whether and when to initiate a new game.
MENACE does not know, nor does it learn, what a game is. The designer of MENACE abstracted that away from the situation, so that MENACE could be a pure learning machine.
That today is both the strength and weakness of modern Machine Learning. Really smart people, researchers or engineers, come up with an abstraction for the problem in the real world that they want to apply ML to. Those same smart people figure out how data should flow to and fro between the learning system and the world to which it is to be applied. They set up machinery which times and gates that information flow from the application. They set up a signaling system on when the learning system is supposed to respond to an input (in MENACE’s case a string of nine characters drawn from ‘.’, ‘X’, and ‘O’) and produce an output. And those same people set up a system which tells the learning system when to learn, to adjust the numbers inside it, in response to a reinforcement signal, or in some other forms of ML a very different, but still similarly abstracted signal–we will see that in the next chapter.
tic-tac-toe machine resonates with modern ML
Although MENACE is well over fifty years old, it it shares many properties with modern Machine Learning systems, though of course it is much smaller and simpler than the systems that people use today–one must expect something from 50+ years of hard intellectual work. But the essential problems that MENACE and today’s ML algorithms have are very instructive as they can give some intuition about some of the limits we might expect for modern AI and ML.
Parameters. After the design work was done on MENACE, all that could change during learning as the value of the 1087 parameters, the numbers of various colored beads in various matchboxes. Those numbers impact the probability of randomly picking a bead of a particular color from a matchbox. If the number of red beads goes down and the number of amber beads goes up over time in a single matchbox, then it is more likely that Alan will pick an amber bead at random. In this way MENACE has learned that for the particular situation on a tic-tac-toe board corresponding to that matchbox the square corresponding to the amber bead is a better square to play than the one corresponding to a red bead. All MEANCE is doing is juggling these numbers up and down. It does not learn any new structure to the problem while it learns. The structure was designed by a researcher or engineer, in this case Donald Michie.
This is completely consistent with most modern Machine Learning systems. The researchers or engineers structure the system and all that can change during learning is a fixed quantity of numbers or parameters, pushing them up or down, but not changing the structure of the system at all. 1087 may seem like a lot of parameters for playing tic-tac-toe, but really that is the price of eliminating the geometry of the board from the MENACE machine. In modern applications of Machine Learning there are often many millions of parameters. Sometimes they take on integer values as do the number of beads in MENACE, but more usually these days the parameters are represented as floating point numbers in computers, things that can take on values like 5.37, -201.65, 894.78253, etc.
Notice how simply changing a big bunch of numbers and not changing the underlying abstraction that connected the external problem (playing tic-tac-toe) to a geometry-free internal representation (the numbers of different colored beads in matchboxes) is very different from how we have become familiar with using computers. When we manage our mail box folders, creating special folders for particular categories (e.g., “upcoming trips”, “kids”, etc.) and then sub folders (e.g., “Chicago May 5”, “soccer”, etc.) and then filing emails in those subfolders, we are changing the structure of our representation of the important things in our life which are covered by emails. Machine Learning, as in the case of MENACE, usually has an engineering phase were the problem is converted to a large number of parameters, and after that there is no dynamic updating of structures.
In contrast, I think all our intuitions tell us that our own learning often has our internal mental models tweak and sometimes even radically change how we are categorizing aspects of the skill or capability that we are learning.
Large Parameters. My computer simulations of MENACE soon had the numbers of beads of a particular color in particular boxes ranging from none or one up to many thousand. This intuitively seem strange but is not uncommon in today’s Machine Learning systems. Sometimes there will be parameters that are between zero and one, were just a change of one ten thousandth in value will have drastic effects on the capabilities that the system is learning, while at the same time there will be parameters that are up in the millions. There is nothing wrong with this, but it does feel a little different from our own introspections of how we might weigh things relatively in our own minds.
Many Examples Needed. If we taught tic-tac-toe to an adult we would think that just a few examples would let them get the hang of the game. MENACE on the other hand, even when carefully tutored by Donald Michie took a couple of hundred examples to get moderately good. My simulation is still making relatively big progress after three thousand games and is often still slowly getting even a little better at four thousand games. In modern Machine Learning systems there may be tens of millions of different examples that are needed to train a particular system to get to adequate performance. But the system does not just get exposed to each of these training examples once. Often each of those millions of examples needs to be shown to the system hundreds of thousands of times. Just being exposed to the examples once leaves way to much bias from the most recently processed examples. Instead by having them re-exposed over and over, after the ML system has already seen all of them many times, the recentness bias gets washed away into more equal influence from all the examples.
Training examples are really important. Learning to play against just one of Player A, B, or C, always lead to very different performance levels against each of these different players with learning turned off in my computer simulation of MENACE. This too is a huge issue for modern Machine Learning systems. With millions of examples needed there is a often a scale issue of how to collect enough training data. In the last couple of years companies have sprung up which specialize in generating training data sets and can be hired for specific projects. But getting a good data set which does not have unexpected biases in it can often be a problem.
When MENACE is trained against Player B, the optimal player that can not be beaten, MENACE does not learn how to win, as it never has an experience of winning so it never receives reinforcement for winning. It does learn how to not be defeated, and so playing against Players A or C its win rate does go up a little as they each sometimes screw up, but MENACE’s winning rate does not go up as much as it does when it trains against those two players. In our example with MENACE my simulations worked best overall when trained against Player C, as that had a mixture of examples that were tough to win against (when it got through a game without making a random bad choice), and because of its occasional random choices examples which more fully spanned all of the possible playing styles MENACE might meet. In the parlance of Machine Learning we would say that when MENACE was trained only against Player B, the optimal player, it overfit its playing style to the relatively small number of games that it saw (no wins, and few losses) so was not capable when playing against more diverse players.
In general, the more complex the problem for which Machine Learning is to be used, the more training data that will be needed. In general, training data sets are a big resource consideration in building a Machine Learning system to solve a problem.
Credit assignment. The particular form of learning that MENACE both first introduced and demonstrates is reinforcement learning, where the system is given feedback only once it has completed a task. If many actions were taken in a row, as is the case with MENACE, either three of four moves of its own before it gets any feedback, then there is the issue of how far back the feedback should be used.
In the original MENACE all three forms of reinforcement, for a win, a draw, or a loss, were equally applied to all the moves. Certainly it makes sense to apply the reinforcement to the last move, as it directly did lead to that win, or a loss. In the case of a draw however, it could in some circumstances not be the best move as perhaps choosing another move would have given a direct win. As we move backward, credit for whether earlier moves were best, worst, or indifferent is a little less certain. In the case of Player A or C as the opponent it may have simply made a bad move in reply to a bad move by MENACE early on, so giving the earlier move three beads for a win may be encouraging something that Player B, the optimal player, will be able to crush. A natural modification would be three beads for the last move in a winning game, two beads for the next to last, and one bead for the third to last move. Of course people have tried all these variations and under different circumstances much more complex schemes would be the best. We will discuss this more, a little later.
In modern reinforcement learning systems a big part of the design is how credit is assigned. In fact now it is often the case that the credit assignment itself is also something that is learned by a parallel learning algorithm, trying to optimize the policy based on the particulars of the environment in which the reinforcement learner finds itself.
Getting front end processing right. In MENACE Michie developed what might be called “front end processing” to map all board positions to only those that were essentially distinct. This simultaneously drastically cut down the number of parameters that had to be learned, let the learning system automatically transfer learning across different cases in the full world (i.e., across symmetries in the tic-tac-toe board), and introduced zero entanglements that could confuse the learning process.
Up until a few years ago Machine Learning systems applied to understanding human speech usually had as their front end programs that had been written by people to determine the fundamental units of speech that were in sound being listened to.
Those fundamental units of speech are called phonemes, and they can be very different for different human languages. Different units of speech lead to different words being heard. For instance, the four English words pad, pat, bad, and bat all have three phonemes with the same middle phoneme corresponding to the vowel sound (in English the same letters may be used represent to different phonemes, so the word paper, while having the same letter ‘a’ for the second phoneme (of four in this word) has a very different sound associated with it, and is therefore a different phoneme), the four different phonemes p, b, d, and t, lead to four different words being heard as p and b are varied at the start, and d and t are varied at the end.
In earlier speech understanding systems the specially built front end phoneme detector programs relied on some numerical estimators of certain frequency characteristics of the sounds and produced phoneme labels as their output that were fed into the Machine Learning system to recognize the speech. It turned out that those detectors were limiting the performance of the speech understanding systems no matter how well they learned. Relatively recently those programs were replaced by other machine learning system, that didn’t necessarily output conventional phoneme representations, and this lead to a remarkable overall increase in reliability of speech understanding systems. This is why today, but only in the last few years, many people now have devices in their homes, such as Amazon’s Echo or Google’s Home, that they can easily interact with via voice.
Getting the front end processing right for an ML problem is a major design exercise. Getting it wrong can lead to much larger learning systems than necessary, making learning slower, perhaps impossibly slower, or it can make the learning problem impossible if it destroys vital information from the real domain. Unfortunately, since in general it is not known whether a particular problem will be amenable to a particular Machine Learning technique, it is often hard to debug where things have gone wrong when an ML system does not perform well. Perhaps inherently the technique being used will not be able to learn what is desired, or perhaps the front end processing is getting in the way of success.
Geometry is hard. Just as MENACE knew no geometry and so tackled tic-tac-toe in a fundamentally different way than how a human would approach it, most Machine Learning systems are not very good at preserving geometry nor therefore are they good at exploiting it. Geometry does not play a role in speech processing, but for many other sorts of tasks there is some inherent value to the geometry of the input data. The engineers or researchers building the front end processing for the system need to find a way to accommodate the poor geometric performance of the ML system being used.
The issue of geometry and the limitations of representing it in a set of numeric parameters arranged in some fixed system, as was the case in MENACE, has long been recognized. It was the major negative result of the book Perceptrons11 written by Marvin Minsky and Seymour Papert in 1969. While people have attributed all sorts of motivations to the authors I think that their insights on this front, formally proved in the limited cases they consider, still ring true today.
Fixed structure stymies generalization. MENACE’s fixed structure meant that anything it implicitly learned about filling or blocking three in a row on a diagonal could not be transferred to filling or blocking a vertical or horizontal row. The fixed structures spanning thousands or millions of variable numerical parameters of most Machine Learning systems likewise stymies generalization. We will see some surprising consequences of this when we look at some of the most recent exciting results in Machine Learning in a later blog post–programs that learn to play a video game but then fail completely and revert to zero capability on exactly the same game when the colors of pixels are mapped to different colorations, or if each individual pixel is replaced by a square of four identical pixels.
Furthermore, any sort of meta-learning is usually impossible too. Since MENACE doesn’t know that it is playing a game, and since there is nothing besides the play and reward mechanism that can access the matchboxes, there is no way that observations of the flow of a game can be ruminated upon. A child might learn a valuable meta-lesson in playing tic-tac-toe, that when you have an opportunity to win take it immediately as it might go away if the other player gets to take a turn. That would correspond to learning rule 1 in our comparison between MENACE and how a person might learn.
Machine Learning engineers and researchers must, at this point in the history of AI, form an optimized and fixed description of the problem and let ML adjust parameters. All possibility of reflective learning is removed from these very impressive learning systems. This greatly restricts how much power of intelligence and AI system with current day Machine Learning systems can tease out of their learning exploits. Humans are generally much much smarter than this.
A Few Developments in Reinforcement Learning
The description of reinforcement learning comes from 1961, and is the first use of the term reinforcement learning when applied to a machine process that I can find. There have been some developments in reinforcement learning since 1961, but only in details as this section shows. The fundamental ideas were all there in Donald Michie’s matchboxes.
Reinforcement learning is still an active field of research and application today. It is commonly used in robotics applications, and for playing games. It was part of the system that beat the world Go champion in 2016, but we will come back to that in a little bit.
After Michie’s first paper, reinforcement learning was formalized over the next twenty years. Without resorting to the mathematical formulation, today reinforcement learning is used where there are a finite number of states that the world can be in. For MENACE those states correspond to the 304 matchboxes of essentially different tic-tac-toe board positions where it is O’s turn to play. For each state there are a number of possible actions (the different colored beads in each matchbox corresponding to the possible moves). The policy that the system currently has is the probability of each action in each state, which for MENACE corresponds to the number of beads of a particular color in a matchbox divided by the total number of beads in that same matchbox. Reinforcement learning tries to learn a good policy.
The structure of states and actions for MENACE, and indeed for reinforcement learning for many games, is a special case, in that the system can never return to a state once it has left it. That would not be the case for chess or Go where it is possible to get back to exactly the same board position that has already been seen.
For many systems of reinforcement learning real numbers are used rather than integers as in MENACE. In some cases they are probabilities, and for a given state they must sum to exactly one. For many large reinforcement learning problems, rather than represent the policy explicitly for each state, it is represented as a function approximated by some other sort of learning system such as a neural network, or a deep learning network. The steps in the reinforcement process are the same, but rather than changing values in a big table of states and actions, the 1087 parameters of MENACE, a learning update is given to another learning system.
MENACE, and many other game playing systems, including chess and Go this time, are a special case of reinforcement learning in another way. The learning system can see the state of the world exactly. In many robotics problems where reinforcement learning is used that is not the case. There the robot may have sensors which can not distinguish all the nuances in the world (e.g., for a flying robot it may not know the exact current wind speed and direction ten meters away from it in the direction of travel). For these sorts of reinforcement learning problems the world is referred to as partially observable.
in MENACE any rewards, be they positive or negative were spread equally over all moves leading up the win, loss, or draw. But in reality it could be that an early move was good, and just a dumb move at the end was bad. To handle this problem Christoper Watkins came up with a method that became known as Q-learning for his Ph.D. thesis12, titled “Learning from Delayed Rewards”, at Cambridge University in 1989. The Q function that he learns is an estimate of what the ultimate reward will be by taking a particular action in a particular state. Three years later he and Peter Dayan published a paper that proved that under some reasonable assumptions his algorithm always eventually converged on the correct answer as to how the reward should be distributed.
This method, which is at its heart the reinforcement learning of Donald Michie’s MENACE from 1961, is what is powering some of today’s headlines. The London company DeepMind, which was bought by Google, uses reinforcement learning (as they explain here) with the Q-learning implemented in something called deep learning (another popular headline topic). This is how they built their Alpha Go program which recently beat both the human Korean and Chinese Go champions.
As a side note, when I visited DeepMind in June this year I asked how well their program would have done if on the day of the tournament the board size had been changed from 19 by 19 to 29 by 29. I estimated that the human champions would have been able to adapt and still play well. My DeepMind hosts laughed and said that even changing to an 18 by 18 board would have completely wiped out their program…this is rather consistent with what we have observed about MENACE. Alpha Go plays Go in a way that is very different from how humans apparatently play Go.
Overloaded words
In English, at least, ships do not swim. Ships cruise or sail, whereas fish and humans swim. However in English planes fly, as do birds. By extension people often fly when they go on vacation or on a business trip. Birds move from one place to another by traveling through the air. These days, so too can people.
But really people do not fly at all like birds fly. Our technology lets us “fly” a quarter of the way around the world, non-stop, in less than a day. Birds who can fly that far non-stop (and there are some) certainly take a lot longer than a day to do that.
If humans could fly like birds we would think nothing of chatting to a friend on the street on a sunny day, and as they walk away, flying up into a nearby tree, landing on a branch, and being completely out of the sun. If I could fly like a bird then when on my morning run I would not have to wait for a bridge to get across the Charles River to get back home, but could choose to just fly across it at any point in its meander.
We do fly. We do not fly like birds. Human flying is very different in scope, in method, and in auxiliary equipment beyond our own bodies.
Arthur Samuel introduced the term Machine Learning for two sorts of things his computer program was doing as it got better and better over time at and through the experience of playing checkers. A person who got better and better over time at and through the experience of playing checkers would certainly be said to be learning to be a better player. With only eight to ten hours experience Samuel’s program (he was so early at this he did not give a name to his program–that innovation had to away the early 1960’s) got better at playing checkers than Samuel himself. Thus, in his first sentence of his paper, again, does Samuel justify the term learning: “The studies reported here have been concerned with programming of a digital computer to behave in a way which, if done by human beings or animals, would be described as involving the process of learning.”
What I have tried to do in this post is to show how Machine Learning works, and to provide an argument that it works in a way that feels very different to how human learning of similar tasks proceeds. Thus, taking an understanding of what it is like for a human to learn something and applying that knowledge to an AI system that is doing Machine Learning may lead to very incorrect conclusions about the capabilities of that AI system.
Minsky13 labels as suitcase words terms like consciousness, experience, and thinking. These are words that have so many different meanings that people can understand different things by them. I think that learning is also a suitcase word. Even for humans it surely refers to many different sorts of phenomena. Learning to ride a bicycle is a very different experience from learning ancient Latin. And there seems to be very little in common in the experience of learning algebra and learning to play tennis. So, too, is Machine Learning very different from any sort of the myriad of different learning capabilities of a person.
The word “learn” can lead to misleading conclusions.
Postscript
I am going to indulge myself a little by pontificating here. Be warned.
In 1991 I wrote a long (I have been pontificating since I was relatively young) paper14 on the history of Artificial Intelligence and how it had been shaped by certain key ideas. In the final paragraphs of that paper I lamented that there was a bandwagon effect in Artificial Intelligence Research, and said that “[m]any lines of research have become goals of pursuit in their own right, with little recall of the reasons for pursuing those lines”.
I think we are in that same position today in regard to Machine Learning. The papers in conferences fall into two categories. One is mathematical results showing that yet another slight variation of a technique is optimal under some carefully constrained definition of optimality. A second type of paper takes a well know learning algorithm, and some new problem area, designs the mapping from the problem to a data representation (e.g., the mapping from tic-tac-toe board positions to the numbers 1 through 304 for the three hundred and four matchboxes that comprise MENACE), and show the results of how well that problem area can be learned.
This would all be admirable if our Machine Learning ecosystem covered even a tiny portion of the capabilities of human learning. It does not. And, I see no alternate evidence of admirability.
Instead I see a bandwagon today, where vast numbers of new recruits to AI/ML have jumped aboard after recent successes of Machine Learning, and are running with particular versions of it as fast as they can. They have neither any understanding of how their tiny little narrow technical field fits into a bigger picture of intelligent systems, nor do they care. They think that the current little hype niche is all that matters, are blind to its limitations, and are uninterested in deeper questions.
I recommend reading Christopher Watkins Ph.D. thesis12 for an example of something that is admirable. It revitalized reinforcement learning by introducing Q-learning, and that is still having impact today, thirty years later. But more importantly most of the thesis is not about the particular algorithm or proofs about how well it works under some newly defined metric. Instead, most of the thesis is an illuminating discussion about animal and human learning, and attempting to get lessons from there about how to design a new learning algorithm. And then he does it.
1 Machine Learning: A Probabilistic Perspective, Kevin P. Murphy, MIT Press, 2012.
2 “Some Studies in Machine Learning Using the Game of Checkers”, Arthur L. Samuel, IBM Journal of Research and Development, 3(3):210–229, 1959.
3 When I first joined the Stanford Artificial Intelligence Laboratory (SAIL) in 1977 I got to meet Arthur Samuel. Born in 1901 he was certainly the oldest person in the lab at that time. After retiring from IBM in 1966 he had come to SAIL as a researcher. Arthur was a delightful and generous person, and besides his research he worked on systems programming in assembler language for the Lab’s time shared computer. He was the principal author of the full screen editor (a rarity at that time) that we had, called Edit TV, or ET at the command level. He was still programming at age 85, and last logged in to the computer system when he was 88, a few months before he passed away.
4 Perhaps I am wrong about exactly what Samuel was referring to. In his Ph.D. thesis12, which I talk about later in the post, Christopher Watkins allows that perhaps Samuel means what I interpret him to mean, though perhaps there is a smarter version of it that was implemented that involved recomputing the saved computations when more of the game tree had been searched. Watkins was unable to tell exactly from reading the paper.
5 “Trial and Error”, Donald Michie, Penguin Science Survey, vol 2, 1961.
6 “How to build a game-learning machine and then teach it to play, and to win”, Martin Gardner, Scientific American, 206(3):138–153, March 1962.
7 We Built Our Own Computers, A. B. Bolt, J. C. Harcourt, J. Hunter, C. T. S. Mayes, A. P. Milne, R. H Surcombe, and D. A. Hobbs, Cambridge University Press, 1966.
8 “Experiments on the Mechanization of Game-Learning Part I. Characterization of the Mode and its parameters”, Donald Michie, Computer Journal, 6(3):232–236, 1963.
9 Michie reports only 287 essentially different situations so his version of MENACE had only 287 matchboxes (though in a 1986 paper he refers to there being 288 matchboxes). Many people have since built copies of MENACE both physically and in computer simulations, and all the ones that I have found on the web report 304 matchboxes, virtual or otherwise. This matches how I counted them in my simulation of MENACE as a program.
10 In all the test results I give I froze the learning and ran 100,000 games–I found that about that number were necessary to give 2 digits, i.e., a percentage, that was stable for different such trials. Note that in total there are 301,248 different legal ways to play out a game of tic-tac-toe. If we consider only essentially different situations by eliminating rotational and reflective symmetries then that number drops to 31,698.
11 Perceptrons: An introduction to Computational Geometry, Marvin Minsky and Seymour Papert, MIT Press, 1968.
12 Learning from Delayed Rewards, Christopher J. C. H. Watkins, Ph.D. thesis, King’s College, Cambridge University, May 1989.
13 The Emotion Machine: Commonsense Thinking, Artificial Intelligence, and the Future of the Human Mind, Marvin Minsky, Simon and Schuster, 2006.
14 “Intelligence Without Reason, Proceedings of 12th International Joint Conference on Artificial Intelligence, Sydney, Australia, August 1991, 569–595.