This is the first post in an intended series on what is the current state of Artificial Intelligence capabilities, and what we can expect in the relative short term. I will be at odds with the more outlandish claims that are circulating in the press, and amongst what I consider an alarmist group that includes people in the AI field and outside of it. In this post I start to introduce some of the key components of my future arguments, as well as show how different any AI system might be from us humans.
Some may recognize the title of this post as an homage to the 1974 paper by Thomas Nagel
, “What Is It Like to Be a Bat?”. Two more recent books, one from 2009 by Alexandra Horowitz
on dogs, and one from 2016 by Peter Godfrey-Smith
on octopuses also pay homage to Nagel’s paper each with a section of a chapter titled “What it is like”, and “What It’s Like”, respectively, giving affirmative responses to their own questions about what is it like to be a dog, or an octopus.
All three authors assume some level of consciousness for the subject animals.
Nagel was interested in the mind-body problem, an old, old, philosophical problem that has perplexed many people since ancient Greek times. Our minds seem to be something very different from the physical objects in our world. How can we understand what a mind is in physical terms (and some deny that there is a physical instantiation), and how is it that this thing so different from normal objects interacts with our body and our environment, both of which seem to be composed of objects?
Nagel says:
Without consciousness the mind-body problem would be much less interesting. With consciousness it seems hopeless.
He then goes on to equate consciousness with there being some way of saying what it is like to have a particular mental state right now. In particular he says:
But fundamentally an organism has conscious mental states if and only if there is something that it is like to be that organism–something it is like for the organism.
He then tries to imagine what it is like to be a bat. He chooses a bat as its perceptual world is so different and alien to our own. In particular at night they “perceive the external world primarily by sonar, or echolocation”, and “bat sonar, though clearly a form of perception, is not similar in its operation and there is no reason to suppose that it is subjectively like anything we can experience or imagine”. Nagel thereby concedes defeat in trying to imagine just what it is like to be a bat. The objective way of looking at a bat externally, does not lead to the subjective understanding that we all have of ourselves. I think that if we ever get to robots with mental states and the robots know about their mental states we will unlikely be able to imagine what it is like to be them. But I think we we can imagine some lesser aspects of being a robot, purely objectively, which will be the main point of this post.
First, however, let’s talk about the same question for dogs and octopuses very briefly, as discussed by the authors of the two books referenced above, Alexandra Horowitz, and Peter Godfrey-Smith.
Godfrey-Smith is fascinated by octopuses and their evolutionarily related cousins cuttlefish and squid. The last common ancestor that their branch of the tree of life had with us, or other mammals, or birds, or fish, or dinosaurs, was about six hundred million years ago. It was a small flat worm, just a few millimeters in size, with perhaps a couple of thousand neurons at most. After the split, our side went on to become vertebrates, i.e., animals with a spine. The other side produced the arthropods including, lobsters, ants, beetles, etc., and a side tree from them turned into the mollusks, which we mostly know as worms, slugs, snails, clams, oysters, scallops and mussels. But a suborder of mollusks is the cephalopods which include the three animals of interest to Godfrey-Smith. His interest is based on how intelligent these animals are.
Clearly these three animals evolved their intelligence separately from the intelligence of birds, lizards, and mammals. Although we can see evolutionarily related features of our brains in brains of very different vertebrates there is no correspondence at all with the brains of these three cephalopods. They have a distributed brain where only a small portion of neurons are in a central location for the brain, rather the majority of the neurons are out in the tentacles. The tentacles appear to autonomously explore things out of sight of the creature, sensing things chemically and by touch, grasping and manipulating small objects or food samples. But if an octopus, in an experimental setting, is required to guide one of its tentacles (through a complex maze, devoid of the chemical signals a tentacle would use on its own) using the vision in its eyes it takes a very long time to do so. At best it seems that the central brain has only supervisory control over the tentacles. They are very different from us, but as we will see later, we can relate to much of the intelligent behavior that they produce, even though on the inside they must indeed be very different. Just as our robots will be very different from us on the inside.
Horowitz studies dogs, and not only imagines what the world seems like to them, but also invokes an idea from Jacob von Uexküll
, a Baltic German biologist who lived from 1864 to 1944. He talked about the Umwelt of an animal, a German word for environment or milieu (literally “around world”) which is the environment in which the animal senses, exists, and acts. An external observer, us humans say, may see a very different embedding of the animal in the world than the animal itself sees. This is going to be true of our robots too. We will see them as sort of like us, anthropomorphizing them, but their sensing, action, and intelligence will make them very different, and very different in the way that they interact with the world.
I have always preferred another concept from von Uexküll, namely Merkwelt
, literally “note world”, which when applied to a creature refers to the world that can be sensed by that creature. It is a part of the Umwelt of a particular creature. The Umwelt of the creature includes the creature itself, the world in which it is, its own Wirkwelt (active world), the effects it can have on the world, and its Merkwelt which is its capability to sense the world. By divorcing the Merkwelt from the creature itself we can talk about it without getting (too) tied up with consciousness.
On the other hand, talking precisely about the Merkwelt of an animal is technically hard to get exactly right. We can observe the Umwelt of an animal, at least using our own Merkwelt. But to understand the Merkwelt of an animal we need to understand what its sensors tell it–and even that is not enough as some sensory values may get to some parts of the brain but not to others. We can look at the physiology of an animal and guess at what sort of things its sensors are telling it, and then set up experiments in the lab where we test the limits of what it might be sensing in those domains. But it is hard to know sometimes whether the animal does not care (in a behavioral sense if we want to leave consciousness out of the picture) about certain sensory stimuli, even if it is aware of them. Then we need to know how the creature processes that sensory data. We will see some examples in humans below, where our own intuitions about how we sense the world, our Merkwelt, are often very wrong.
For robots it is a different story. We build the robots! So we know what sensors we have put in the robot, and we can measure the limits of those sensors and what will make a digital bit flip in the output of the sensors, or not. And we can trace in the wiring and the code where those sensor values are sent throughout the whole mechanical, electrical, and computational robot. In theory we can have complete access to the Merkwelt of a robot. Something we can hardly do at all for the Merkwelt of an animal (or even, really, for ourselves). However, some of the AI techniques we might use in robots introduce new levels of uncertainty about the interpretation of the Merkwelt by the robots–they are not as transparent as we might think, and soon we will be sliding back to having to worry about the complete Umwelt.
In this post I am going to talk about the possible Merwelts (or Merkwelten) of robots, first by talking about the Merkwelts of some animals, and how different they are from our own human Merkwelt, and then extending the description to robots. And taking Nagel’s advice I am going to largely remove the notion of consciousness from this discussion, in contrast to Nagel, Horowitz, and Godfrey-Smith. But. But. Consciousness keeps slipping its way in, as it is the only way we have of thinking about thinking. And so first I will talk about consciousness a little, trying to avoid toppling over the edge to the grease covered philosophical slope that slides away unendingly for most who dare go there at all.
Some COMMENTS ON CONSCIOUSNESS in animals
What consciousness is is mysterious. Some have claimed that it might be a fundamental type in our universe, just as mass, energy and time are fundamental types. I find this highly unlikely as for all other fundamental types we see ways in which they interact. But we do not see any sign of psychokinesis events (outside of movies), where a consciousness has some impact directly on some other thing, outside of the body containing the consciousness. I remain a solid skeptic about consciousness as a fundamental type. I think it is an emergent property of the way a particular animal is put together, including its body and nervous system.
So let’s talk about who or what has it. We can only be absolutely sure that we ourselves have it. We are each sure we do, but it is hard to be sure that other humans do, let alone animals. However it seems highly unlikely that you, the reader, would be the one human being in the world with consciousness, so most likely you will grudgingly admit that I must have it too. Once we assume all humans have consciousness we are confronted with a gradualism argument if we want to believe that only humans have it. Could it really be that humans have had consciousness and that Neanderthals had none? Humans interbed with Neanderthals and most of us carry some of their DNA today. Were they just Stepford wives and husbands to humans? And if Neanderthals had it then probably many other hominoid species had it. And if them, how about the great apes? Look at this orangutan’s reaction to a magic trick and ask could it be that this being has no sense of self, no subjective experiences, nothing at all like what us humans feel? So I think we have to admit it for orangutans. Then how far does it extend, and in what form?
Could octopuses have anything like consciousness? They evolved separately from us and have a very different brain structure.
Octopuses can be trained to do many things that vertebrate animals in laboratories can be trained to do, to solve mazes, to push levers for food, to visually recognize which of two known environments it has been placed in and respond appropriately, etc. More interesting, perhaps, are the very many anecdotal stories of observed behaviors, often in many different laboratories around the world. Octopuses are known to squirt water from their tanks at specific people, drenching them with up to half a gallon of water in a single squirt. In some instances it has been a particular octopus regularly attacking a particular person, and in another instance, a cuttlefish attacking all new visitors to the lab. Thus octopuses react to people as individuals, unlike the way ants react to us, if at all. It seems that octopuses are able to recognize individual humans over extended time periods. But more than that, the octopuses are masters of escape, they can often climb out of their tanks, and steal food not meant for them. And in at least one lab the octopuses learned to squirt water at light bulbs, shorting them out. Lab workers repeatedly report that the escape, theft, or destruction of lighting, happens without warning just as the person’s attention wanders from the octopus and they are looking in another direction–the octopus can apparently estimate the direction of a person’s gaze both when the person is wearing a scuba mask underwater or is unencumbered on land. People who work with octopuses see intelligence at work. In the wild, Godfrey-Smith reports following individual octopuses for 15 minutes as they wander far and wide in search of food, and then when they are done, taking a direct route home to their lair. And he reports on yet another experimenter saying that unlike fish, which just exist in a tank in captivity, the behavior of captive octopuses is all about testing the limits of the tank, and they certainly appear to the humans around them to well know they are in captivity.
But are all these observations of octopuses an indication of consciousness?
Nagel struggles, of course, with defining consciousness. In his bat paper he tries to finesse the issue be inventing the term subjective experience as a key ingredient of consciousness, and then talking about those sorts of experiences. By subjective experience Nagel means what it feels like to the creature which has that experience. An outsider may well observe the objective reality of the creature having that experience, but not do so well at understanding how it feels to the creature.
For us humans we can mostly appreciate and empathize with how an experience that another human has is felt. We know what it feels like for ourself to walk through a large room cluttered with furniture, and so we know what it feels like to another person. Mostly. If we are sighted and the other person is blind, but they are clicking their tongue to do echo location it is much harder for us to know how that must feel and how they experience the room. As we get further away from ourselves it becomes very hard for us to even imagine, let alone know, what some experience feels like to another creature. It eventually becomes hard to make an objective determination whether it feels like anything at all to the creature.
Each of Nagel, Horowitz, and Godfrey-Smith argue for some form of subjective experience in their subject animals.
Godfrey-Smith makes a distinction between animals that seem to be aware of pain and those that do not. Insects can suffer severe injuries and will continue trying to do whatever task they are involved in with whatever parts of their bodies are still functional and without appearing to notice the injury at all. In contrast, an octopus acts jumps and flails when bitten by a fish, and for more severe injuries pays attention to and is protective of the injured region. To Godfrey-Smith this is a threshold for awareness of pain. He points out that zebrafish who have been injected with a chemical that is suspected of causing pain, will change their favored choice of environment to one that contains painkiller in the water. The zebrafish makes a choice of action in order to reduce its pain.
But is it also the ability to remember episodes (called episodic memory), past experiences as sequences of events, involving self, and to deduce what might happen next from those? Or is it the ability to build mental maps of the environment, and now where we are placed in them, and plan how to get to places we want to go? Is it a whole collection of little things that come together to give us subjective experiences? If so, it does seem that many animals share some of those capabilities with us humans.
Dogs seem to have some form of episodic memory as they go to play patterns from the past with people or dogs that they re-encounter after many years, they pick up on when they are being walked or driven to some place that they do not like (such as the vet’s) and they pick up on behaviors of their human friends and seem to predict what their near term future emotional state is going to be as the human continues their established sequence of behaviors on the world. E.g., a dog might notice when their human friend is engaging in an activity that we would label as packing their bag for a trip, and go to the emotional state of being alone and uncomforted by that human’s presence.
On the other hand, dogs do not realize that it is them when they see themselves in a mirror, unlike all great apes (including us humans!) and dolphins. So they do not seem to have the same sense of self that those animals have. But do they realize that they have agency in the world? While they are not very good at hiding their emotions, which clearly they do have, they do have a sort of sense of decorum about what behaviors to display in front of people. A dear dog of mine would never ever jump up on to a table. She would get up on to chairs and couches and beds, but not tables. When our gardener was around mowing the lawn in a circle around the house she would run from glass door to glass door, very upset and barking, watching him make progress on each loop around the house, anticipating where he would appear next (more episodic memory perhaps!). And then one day Steve told us that when we where not home and he was mowing the lawn she would always jump up on the kitchen table, barking like crazy at him, watching has he moved from the east side to the south side of the house. She knew not to do that in front of us, but when we were not around her emotions were no longer suppressed and they got the better of her. That seems like a subjective experience to me.
Overall dogs don’t seem to exhibit the level of consciousness that we do, which is somehow wrapped up with subjective experiences, a sense of self, an ability to recall episodes in our lives, and to perhaps predict how we might feel, or experience, should a certain thing happen in the future.
I am pretty sure that no AI system, and no robot, that has been built by humans to date, possesses even a rudimentary form of consciousness, or even one that have any subjective experience, let alone any sense of self. One of the reasons for this is that hardly anyone is working on this problem! There is no funding for building conscious robots, or even conscious AI systems in a box for two reasons. First, no one has elucidated a solid argument that it would be beneficial in any way in terms of performance for robots to have consciousness (I tend to cautiously disagree–I think it might be a big breakthrough), and second, no one has any idea how to proceed to make it so.
Later in this post I refer to the SLAM problem (about building maps) which has been a major success in robotics over the last thirty years. That was not solved by one person or one research group. Real progress began when hundreds of researchers all over the globe started working on a commonly defined problem, and worked on it as a major focus for almost 20 years. At the moment for conscious robots we only have a small handful of researchers, no common definition or purpose, and no real path forward. It won’t just happen by itself.
some comments on unconsciousness in Humans
As we have discussed above, while animals have some aspects of consciousness we have seen no real evidence that they experience it as vividly as do we. But before we get too high horsey about how great we are, we need to remember that even for humans, consciousness may be a blatant luxury (or curse) that we may not really need for who we are.
Godfrey-Smith relates the case of a woman who can see, but who has no conscious experience of being able to see. This situation is known as blindsight although Godfrey-Smith does not seem to be aware that this phenomenon had previously been discussed with earlier patients, in a very scholarly book titled “Blindsight”
. We will stick with Godfrey-Smith’s case as he has produced a succinct summary.
The patient is a woman referred to as DF who had damage to her brain from carbon monoxide poisoning in 1988.
As a result of the accident, DF felt almost blind. She lost all experience of the shapes and layout of objects in her visual field. Only vague patches of color remained. Despite this, it turned out that she could still act quite effectively toward the objects in space around her. For example, she could post letters through a slot that was placed at various different angles. But she could not describe the angle of the slot, or indicate it by pointing. As far as subjective experience goes, she couldn’t see the slot at all, but the letter reliably went in.
Godfrey-Smith goes on to report that even though she feels as though she is blind she can walk around obstacles placed in front of her.
The human brain seems to have two streams of visual processing going through two different parts of the brain. There is one that is used for real-time bodily adjustments as one navigates through space (walking, or getting the letter through the slot). That area of the brain was undamaged in DF. The other area, where vision is used for categorization, recognition, and description of objects was the part that was damaged in DF, and with that gone so went the subjective experience of seeing.
We all know that we are able to do many things unconsciously, perhaps more than we are conscious of! Perhaps we are only conscious of some things, and make up stories that explain what we are doing unconsciously. There are a whole class of patients with brains split at the corpus callosum, where this making stuff up can be readily observed, but I will save that for another post.
A Short Aside
Apart from the main content of this post, I want to point out one, to me, miraculous piece of data. We now can see that intelligence evolved not once, but at least twice, on Earth.
The fact that a bilateral flatworm is the common ancestor of two branches of life, the vertebrate branch and another that in turn split into arthropods and mollusks is very telling. Today’s closest relatives to those old flatworms from 600 million years ago, such as Notoplana Acticola, have about 2,000 neurons. That is far less than many of the arthropods have (100,000 is not uncommon for insects), and it seems that insects have no subjective experience. It is extremely unlikely, therefore, that the flatworms were anything more than insect like.
That means that the intelligence of octopuses, and other cephalopods, evolved completely independently from the evolution of the intelligence of birds and mammals. And yet, we are able to recognize that intelligence in their behavior, even though they have very alien Merkwelts compared to us.
Intelligence evolved at least twice on Earth!
So far we have observed 100 billion galaxies with the Hubble Space Telescope. It is expected that when the Webb Space Telescope gets into orbit we will double that number, but let’s be conservative for now with what we have seen. Our own galaxy, which is very typical has about 100 billion stars. Our Sun is just one of them. So we know that there are 10,000,000,000,000,000,000,000 stars out there, at least. That is
stars. It is estimated that there are
grains of sand on Earth. So for every grain of sand, on every beach, and every reef in the whole Earth, there are more than 1,000 stars in our Universe.
In the last 20 years we have started looking for planets around stars in our very tiny local neighborhood of our galaxy. We have found thousands of planets, and it seems that every star has at least one planet. One in six of them has an Earth sized planet. In February of this year NASA’s Spitzer Space Telescope found that a star only 40 light years from Earth (our galaxy is 100,000 light years across, so that is really just down the street, relatively speaking) has seven Earth sized planets, and three of them are about the same temperature as Earth, which is good for Earth-like life forms.
See my blog post on how flexible it turns out even Earth-based DNA is and how we now know that living systems do not need to have exactly our sort of DNA. Enormous numbers of planets in the Universe, and just our local galaxy, with still enormous numbers of Earth like planets, and lots of routes to life existing on lots of those planets. And in the one instance we know about where life arose we have gotten intelligence from non-intelligence at least twice, totally independently. This all increases my confidence that intelligent life is abundant in the Universe. At least as intelligent as octopuses, and birds, and mammals, and probably as intelligent as us. With consciousness, with religions, with Gods, with saviors, with territorialism, with hate, with fear, with war, with grace, with humor, with love, with history, with aspirations, with wonder.
There is a lot of exciting stuff out there in the Universe if we can figure out how to observe it!!!!
THE MERKWELTEN OF OTHER ANIMALS
Let’s now talk about the Merkwelten (plural of Merkwelt) of octopuses, dogs, and humans. Much, but certainly not all, of the material below on octopuses and dogs is drawn from the books of Godfrey-Smith and Horowitz. By comparing these three species we’ll be attuned to what to think about for the Merkwelt of a robot.
Octopuses are able to respond to visual stimuli quite well, and it seems from the anecdotes that they can see well enough to know when a person is looking at them or looking away. There is good evolutionary sense in understanding whether potential predators are looking at you or not and even some snakes can exhibit this visual skill. But octopuses can be trained on many tasks that require vision. Unlike humans, however, the training seems to be largely eye specific. If an octopus is trained on a task with only one of its eyes being able to see the relevant features, it is unable to do the task using its other eye. Humans, on the other hand, unless their corpus callosum has been severed, are able to effortless transfer tasks between eyes.
Godfrey-Smith recounts a 1956 experiment with training octopuses to visually distinguish between large squares and small squares, and behave differently to get a reward (or to avoid a shock…it was 1956, well before octopuses were declared honorary vertebrates for the purpose of lab animal rules) for the two cases. The octopuses were able to make the right distinctions independent of how far away the squares were placed from them–a small square placed close to an octopus would appear large in its visual field, but the octopuses were able to correct for this and recognize it correctly as a small square. This tends to suggest that the squares are more than just visual stimuli. Instead they are treated as objects independent of the location in the world, and the octopus’s point of view. This feels like the square is a subjective experience to the octopus. This capability is known as having perceptual constancy, something not seen in insects.
Octopuses are also very tactile. They reach out their tentacles to feel new objects, and often touch people with a single tentacle. Besides touch it is thought that they are able to taste, or smell, with the tentacles, or at least sense certain molecules. Eight touchy feely tasting sensors going in all directions at once. This is a very different Merkwelt from us humans.
Another astonishing feature of octopuses, and even more so of cuttlefish, is their ability to rapidly change their skin color in very fine detail. They have a number of different mechanisms embedded in their skin which are all used in synchrony to get different colors and patterns, sometimes rippling across their skin much like a movie on a screen. They are able to blend in to their surroundings by mimicking the color and texture around them, becoming invisible to predators and prey alike.
But here is the kicker. Both octopuses and cuttlefish are color blind! They only have one sort of visual receptor in their eyes which would predict color blindness. And there have been no tests found where they respond to different colors by seeing them with their eyes. Their skin however seems to be color sensitive and that programs what is shown on the other side of a tentacle or the body, in order to camouflage the octopus. So octopuses have blindsight color vision, but not via their eyes. They can make no decisions at a high level based on color, but their bodies are attuned to it.
Is this so very different to us, in principle? Our gut busily digests our food, responding to what sort of food is there, and no matter how hard we think, we can neither be aware of what details have been detected in our food (except in the rare case that it causes some sort of breakdown and we vomit, or some such), nor can we control through our thoughts the rate at which it is digested. Like the octopus, we too have autonomous systems that do not rise to the level of our cognition.
So let’s bring all this together and see if we can imagine the Merkwelt of an octopus. Let’s be an octopus. We know the different feeling of being in water and out of water, and when we see places that are out of water we know they are non-watery. We see things with one eye, and different things with another eye, but we don’t necessarily put that those two views together into a complete world view. Though if we are seeing things that have permanence, rather than being episodic, we can put them into a mental map, and then recognize them with either eye, and into our sense of place as we wander around. We rarely see things upside down, as our eyes rotate to always have the same orientation with respect to up and down, almost no matter what our bodies are doing, so we see the world from a consistent point of view. Unfortunately what we see is without color, even in the middle of the day, and near the surface. We can only hear fairly low frequency sounds, but that is enough to hear some movements about us. In contrast to our dull sight and hearing, the world mediated via our tentacles is a rich one indeed. We have eight of them and we can make them do things on command, but if we are not telling them exactly what to do they wander around a bit, and we can smell and feel what they are touching. We can smell the water with our tentacles, smell the currents going by, and smell the things our tentacles touch. It is as rich a sense of the being in the world as seeing it is for many other animals.
Let’s now turn to dogs. We all know that their sense of smell is acute, but first let’s look at their eyesight.
The eyes of mammals have two different sorts of light receptors. Cones are color sensitive, and rods, which work in much dimmer light are not, and that is why we lose our color sensitivity at night–we are using different sensor elements to get much of our visual information. Humans have three sorts of cones, each sensitive to a different part of the color spectrum. The following is a typical human sensitivity profile, taken from Wikimedia.
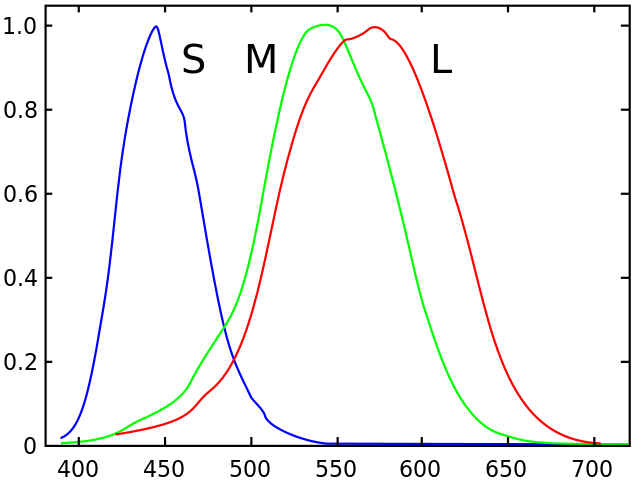
The longer wavelength version is sensitive to colors centered in the red area of the spectrum (the bottom axis is giving the wavelength of light in nanometers), the middle wavelength around green, and the shorter one is centered around blue. They all overlap and there is quite a bit of processing to get to a perceived color–we’ll come back to that below. Just as individual humans have physical variations in their bone lengths, muscle mass, etc., so too do individuals have slightly different shaped sensitivity curves for their cones, and different values for peak sensitivity. So we probably all perceive colors slightly differently. Also it turns out that about 12% of woman have a fourth type of cone, one centered on yellow, between red and green. However, for very few of those women is the cone’s peak sensitivity far enough away from their red peak to make much difference–for the few where it is much displaced, a much richer color palette can be perceived than for most people. Those few have a quite different color Merkwelt than the rest of us.
Dogs, on the other hand have only two sorts of cones, but many more rods. Their night vision is much better than ours, though their acuity, the ability to distinguish fine detail, is less than ours. Their color cones are centered on yellow and blue, and recent evidence suggests that they can see down into the ultraviolet with their blue cones, at much shorter wavelengths than we can see at all. So they are certainly not color blind but they see different colors than we do. Since the color that we see for an object is often the blend of many different materials in the object, reflecting light at different wavelengths, there are surely materials in objects that we see as some particular color, that a dog sees as a very different color. So our beautiful beige bathroom where the floor tiles blend with the shower stall tiles, and blend with the color of the paint on the walls, may instead be a cacophony of different colors to a dog. We won’t know if our dog is seeing our bathroom or living room more like this:
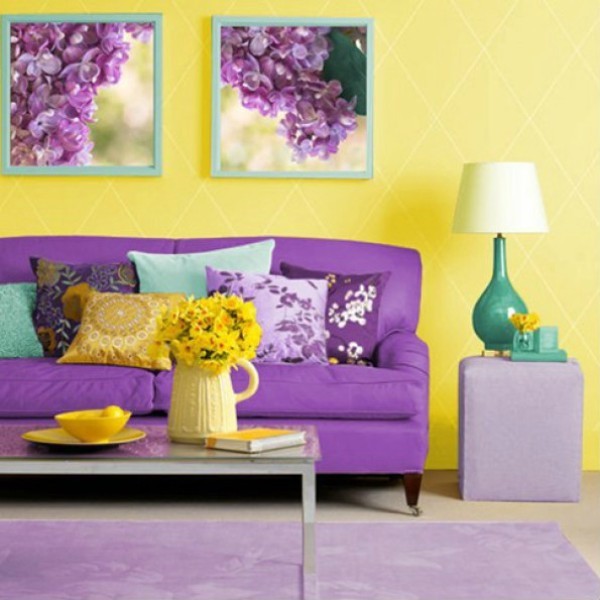
where we might be seeing it as a blend of soothing colors. Beyond the direct sensory experience we don’t know if dogs have any developed taste for what is a calm set of colors and what is a jarring set of colors.
What we do know about dogs is that they are not able to distinguish green and red, and that different electric light spectra will alter what they see in the world in ways different to how it alters things for us. If you have seen blue LED street lights you will know just how much different lighting can change our perceptions. The same is true for dogs, but in different ways that are hard for us to appreciate how it might feel to them. But, I am pretty sure that dogs whose owners dress them up in red and green for Christmas are not having the festive nature of their clothes seep into their doggy consciousness…to them it is all gray and grey.
The big part of a dog’s Merkwelt, to which we are largely not privy at all, is through its sense of smell. We have all seen how dogs sniff, and push their nose into things, lift their nose into the air to catch scents on the breeze, and generally get their nose into everything, including butts, crotches, and the ground.
Horowitz estimates that a dogs sense of smell is a million times more sensitive than ours–it is hard for us to imagine that, and feels overwhelming compared to our rather little used noses. She points out that to a dog every petal of a single flower smells different, and the history of what insects have been there is all there in the dog’s Merkwelt. More than just smelling things as we might, dogs can tell how the smell is changing in just a few seconds, giving them time information that we have never experienced.
To a dog the world is full of smells, laid down weeks and seconds ago, distinguishable by time, and with information about the health and activities of people, animals, and plants. Dogs may not react differently to us when they see us naked or dressed, but they do react differently by detecting where we have been, what we have been doing, and whether we are ill–all through smell.
And just as our eyesight may reveal things that dogs may not notice, dogs notice things that we may be oblivious to. Horowitz points out that the new dog bed we buy might smell terrible to a dog, just as the purple and yellow living room above looks atrocious to me. A dog’s Merkwelt is very different from ours.
The Merkwelt of Humans
We will get to robots soon. But we really need to talk about the Merkwelt of humans first. Our own subjective experiences tell us that we perceive the world “as it is”. But since bats, octopuses, and dogs perceive the world as it is for them, perhaps we are not perceiving any particular ground truth either!
Donald Hoffman at the University of California at Irvine (previously he was at the AI Lab in 1983 just as he finished up his Ph.D. at M.I.T.), has argued for a long time that our perceptual world, our Merkwelt is not what the real world is at all. His 1998 book “Visual Intelligence: How We Create What We See”, makes this point of view clear in its title. A 2016 article and interview with him in The Atlantic is a very concise summarization of his views. And within that I think this quote from him gets at the real essence of what he is saying:
Suppose there’s a blue rectangular icon on the lower right corner of your computer’s desktop — does that mean that the file itself is blue and rectangular and lives in the lower right corner of your computer? Of course not. But those are the only things that can be asserted about anything on the desktop — it has color, position, and shape. Those are the only categories available to you, and yet none of them are true about the file itself or anything in the computer. They couldn’t possibly be true. That’s an interesting thing. You could not form a true description of the innards of the computer if your entire view of reality was confined to the desktop. And yet the desktop is useful. That blue rectangular icon guides my behavior, and it hides a complex reality that I don’t need to know. That’s the key idea. Evolution has shaped us with perceptions that allow us to survive. They guide adaptive behaviors. But part of that involves hiding from us the stuff we don’t need to know. And that’s pretty much all of reality, whatever reality might be.
By going from the natural world to the world of the computer (this back and forth is going to get really interesting and circular when we do finally get to robots) he is able to give a very convincing argument about what he means. And then we humans need to extend that argument to the world we normally inhabit by analogy, and we start to see how this might be true.
I, and many others, find the world of so-called “optical illusions” to be very helpful in convincing me about the truth of the disconnect between human perception, our Merkwelt, and the reality of the Universe. Perhaps the greatest creator of images that show us this disconnect is Akiyoshi Kitaoka from Ritsumeikan University in Kyoto, Japan.
If you go to his illusion page you will see static images that appear to move, straight lines that bulge, waves made from 2D squares, and concentric circles that appear to be spirals, and links to almost 60 pages of other illusions he has created.
But the one of strawberries reproduced here is perhaps the best:
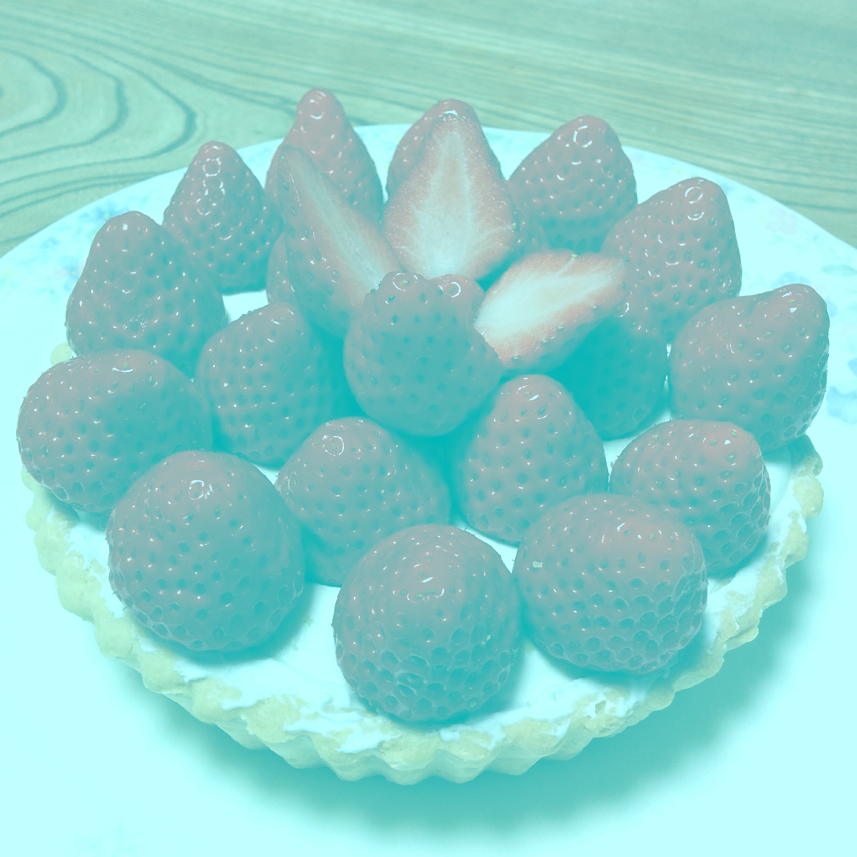
Our perceptual system sees red strawberries. But there are none in the image. There are no red pixels in the image. Here is the tip of the bottom center strawberry expanded.

If you think you see redness there cover up the bottom half with your hand, and it looks grey. Or scroll it to the top of the screen so that the strawberry image is out of view. For me, at least, as I scroll quickly past the strawberry image, the redness of the strawberries temporarily bleeds out into the grey background on which the text is set!
A pixel on a screen is made up of three different colors, red, green, and blue, usually where each of R, G, and B, are represented by a number in the range 0 to 255, to describe how bright the red, green, or blue should light up at a particular point of the screen or image, where 0 means none of that color and 255 means as much as possible. In looking through the raw 734,449 pixels in the strawberry image, only 122, or 0.017%, have more red than each of green and blue at a pixel, and the biggest margin is only 6 out of 255. Here are the three such colors, with the most more red than both green and blue, just duplicated to make a square. All grey!
The values of those RGB pixels, left to right are (192, 186, 186) = 0xc0baba, (193, 187, 187) = 0xc1bbbb, and (189, 183, 183) = 0xbdb7b7. All the pixels that are more red than both green and blue look grey in isolation. The right hand square represents the most red pixel of all in the picture, and perhaps it looks a tinge of red, nothing like the redness we see in the strawberries, and only a tiny handful of pixels are this red in the image. In fact there are only 1,156 pixels, or 0.16%, where red is not the smallest component of the color.
The most red in any of the pixels has a value of 195, but in all of them there is more of one of the other colors, green or blue. Here are three of the pixel colors, from 18 total colors, in the image that have the most red in them (values 0xc3ffff, 0xc3e5ef, and 0xc3c4be):
But the image looks to us humans like it is a picture of red strawberries, with some weird lighting perhaps, even though the pixels are not red in isolation.
We have our Merkwelt which is our sensor world, what we can sense, but then our brain interprets things based on our experience. Because we might come across strawberries under all sorts of different lighting conditions it makes sense for us to not simply rely on the raw color that we perceive. Instead we take lots of different clues, and map a whole lot of greys and cyans, with some contrast structure in them, into rich red strawberries. In this case our perceptual system gets it right. Some of Professor Kitaoka’s images show us how we can get it wrong.
So… bats and dogs probably do some of this too, perhaps in echo space or in smell space. Octopuses most likely also do it. Will our robots need to do it to be truly effective in the world?
THE MERKWELT OF TODAY’s DOMESTIC ROBOTS
The only mass deployed robots in the home today are robot vacuum cleaners. A company I cofounded, iRobot, though not the first to market, lead the way in getting them out into the world, and it alone has sold over 20 million Roombas. Other companies, both original technology developers, and illegal copiers (sometimes on iRobot tooling) in China have also joined the market, so there may well be 50 million of these robots worldwide now.
The early version robot vacuum cleaners had very few sensors, and a very simple Merkwelt. They had bump sensors, redundant cliff sensors, a sensor for infrared beams, and a voltage sensor on its recharging contacts. The bump sensor simply tells the robot it hit something. The cliff sensors, a downward looking infrared “radar”, and a wheel drop sensor, tell the robot when it is at a drop in the floor. Two types of infrared beams were sensed, one a virtual wall that a home owner could set up as a no go line in their house. The early Roombas also always had two internal sensors, one for its own battery voltage so that it could know when recharging was needed, or complete. If the battery voltage was down it would stop cleaning and wander in search of a special infrared beacon that it could line up on and go park itself on the recharging unit. There was also one for when the dirt bin was full, and that too would trigger the go-park-at-home behavior, though in the very earliest version before we had recharging stations it would just stop in its tracks, and play a little tune indicating that the bin was full and shut down
.
These Roombas were rather like Godfrey-Smith’s discussion of insects. If some part of them failed, if one of the drive wheels failed for instance, they just continued on blissfully unaware of their failure, and in this case ran around in a circle. They had no awareness of self, and no awareness that they were cleaning, or “feeding”, in any sense; no sense or internal representation of agency. If they fed a lot, their bin full sensor would say they were “satiated”, but there was no connection between those two events inside the robot in any way, whether physically or in software. No adaptation of behavior based on past experience. Even the 2,000 neuron flatworms that exist today modify their behaviors based on experience.
Physics often comes into play when talking about robots, and there was one key issue of physics which has an enormous impact on how Roombas clean. They are small and light, and need to be for a host of reasons, including being safe should they run into a sleeping dog or baby on the floor, and not stressing a person bending down to put them on the floor. But that means they can not have much onboard battery, and if they were designed to vacuum like a typical vacuum cleaner the 300 Watts of power needed to suck up dirt from carpets would limit the battery life to just a very few minutes. The solution was to have a two stage cleaning system. The first stage was two counter rotating brushes lateral across the body perpendicular to the direction of travel. That pulled up dirt near the surface and managed to collect the larger particles that it it threw up. Following that was a very thin linear slit, parallel to the brushes, sucking air. By being being very thin it could achieve suction levels similar to those of a 300 Watt vacuum cleaner with only 30 Watts. But it could not actually suck any but very small particles through the orifice. But indeed, that is how it cleans up the fine dust, but leaves larger particles on the top of the carpet. And that is why the Roomba needs to randomly pass over a surface many times. The next time around those larger particles will already be on the surface and the brushes will have a better chance to getting them up.
A side effect of those powerful brushes is that tassels of rugs, or power cords lying on the floor would get dragged into the brush mechanism and soon would be wrapped around and around, leaving the robot straining in place, with an eventual cut off due to perceived high current drain from the motors. But the early versions were very much like insects, not noticing that something bad was happening, and not modifying their behavior. Later versions of the Roomba solved this problem by noticing the load on the brushes as something tangled, and reversing the direction of the brushes backing the tangler out. But still no subjective experience, still no noting of the location of a tassel and avoiding it next time through that area–even very simple animals have that level of awareness.
Another problem for the first decade of Roombas was that brushes would eventually get full of knotted air, reducing their efficiency in picking up dirt. Despite the instructions that came with the robot most people rarely cleaned the brushes (who would want to?–that’s why you have a robot vacuum cleaner, to do the dirty work!). Later versions clean the brushes automatically, but again there is no connection between that behavior and other observations the robot might make from its Merkwelt.
Well before the bush cleaning problem was solved there was a sensor added internally to measure how much dirt was being picked up. If there was a lot, then the robot would go into a circling mode going over the same small area again and again as there was most likely a whole lot of dirt in that area. Again, no awareness of this was connected to any other behavior of the robot.
More recently Roomba’s have added a sense of place, through the use of an upward looking camera. As the robot navigates it remembers visual features, and along with a crude estimate of how far it has travelled (the direction of the nap of a carpet can induce 10% differences in straight line odometry estimate in one direction or the other, and turns are much worse), it recognizes when it is seeing the same feature again. There has been thirty years of sustained academic work on this problem, known as SLAM (Simultaneous Localization And Mapping), and Roomba has a vision based version of this–known as VSLAM. The Roomba uses the map that it creates to check off areas that it has spent time in so that it is able to spread out its limited cleaning time. It also allows it to go in straight lines as customers seem to want that, as though they are not aware of the way they, humans, vacuum a room, with lots of back and forth motions, and certainly no straight line driving the vacuum cleaner up and back along a room.
VSLAM is as close as a Roomba gets to episodic memory, but it is not really generalized episodes, it doesn’t know about other things and incidents that happened relative to that map. It seems a much weaker connection to the geometry of the world than birds or mammals or octopuses have.
It is plausible given the Merkwelt of recent Roombas, those with a camera for VSLAM, that some sort of sense of self could be programmed, some some sort of very tenuous subjective experience could be installed, but really there is none. Will people building the next generation of domestic robots, with more complex Merkwelts, be tempted, able, or desirous of, building robots with subjective experiences. I think that remains to be seen, but let’s now look at where technology market forces are going to drive the Merkwelt of domestic robots, and so drive what it will be like to be such a robot.
The Merkwelt OF TOmoRROW’S Domestic Robots
There are already more robot vacuum cleaners in people’s homes than many species of intelligent animals have in absolute numbers. Their Merkwelt is not so interesting. But there are lots and lots of startups and established companies which want to built domestic robots, as there is a belief that this is going to be a big growth area. I believe that too, and will be talking about how the world-wide demographic inversion will drive that, in a soon to be finished blog post. This next generation of domestic robots is going to have a the possibility of a much richer raw Merkwelt than previous ones.
The new Merkwelt will be largely driven by the success of smart phones over the last ten years, and what that has done to the price and performance of certain sensors, and to low power, physically small computation units. And this Merkwelt can be very different from that of us, or dogs, or octopuses, or bats. It is going to be very hard, over the next few paragraphs, for us to think about what is it like to be one of these robots–it will stretch us more than thinking about our relatively close relatives, the octopuses.
The innards of a modern smart phone, without the actual cameras, without the expensive touch screen, without the speakers and microphone, without the high performance batteries, and without the beautifully machined metal case, are worth at retail about $100 to $200. Both Samsung and Qualcomm, two of the biggest manufacturers of chips for phones, sell boards at retail, in quantity of one, which have most of the rest of a modern cell phone for about $100. This includes eight high performance 64 bit processors, driver circuitry for two high definition cameras, the GPU and drivers for the screen, special purpose silicon that finds faces so that the focus in photos can be on them, special purpose speech processing and sound generating silicon, vast quantities of computer memory, cryptographic hardware to protect code and data from external attacks, and WiFi and Bluetooth drivers and antennas. Missing is the GPS system, the motion sensors, NFC (near field communication) for things like Apple Pay, and the radio frequency hardware to connect to cellular networks. Rounding up to $200 to include all those is a safe bet.
Anyone considering building a domestic robot in the next five years would be crazy not to take advantage of all this phone capability. $200 in quantity of one gets a vast leg up on building the guts of a domestic robot. So this is what will largely drive the Merkwelt of our coming domestic robots over the next decade or so, and phones may continue to drive it, as phones themselves change, for many decades. Don’t be surprised to see more silicon in phones over the next few years that is dedicated to the runtime evaluation of deep learning (see my recent post on the end of Moore’s Law).
There may well be other sensors added to domestic robots that are not in phones, and they will be connected to the processors and have their data handled there, so that will be added to the Merkwelt. But a lot of Merkwelt is going to come from the cameras and microphones, and radio spectra of mobile phones.
In the forthcoming 5G chip sets for mobile phones there will be a total of nine different radio systems on high end smart phones.
Even if only equipped with the $100 stripped down phone systems our domestic robots will be able to “smell” our Bluetooth devices and our WiFi access points, and any devices that use WiFi. As I look around my house I see WiFi printers, laptops, tablets, smart phones, and a Roku device attached to my TV (more recent “smart TVs” have WiFi connections directly). As active Bluetooth devices I have computer mice, keyboards, scales, Fitbits, watches, speakers, and many more. The Merkwelt of our domestic robots will include all these devices. Some will be in fixed locations, some will move around with people. Some will be useful for associating with a particular person who might also be located by cameras on the robot. But the robot will have eyes in the back of its head–without pointing its cameras in some direction it may well be able to know when a particular person is approaching just from their Bluetooth signature. With this Merkwelt, and just a little bit of processing, our domestic robots can have a totally different view of the world than we have–a technological view provided by our communication devices. To us humans they are just that, communication devices, but to our robots they will be geographic and personal tags, understood by viewing just a few identifying bits of information within the signals. But depending on who builds the robots and who programs them they might be able to extract much more information about us than just distinguishing us as one that has been seen before. Perhaps they will have the ability to listen in on the content of our communications, be it how many steps we have taken today, or the words we are typing to our computer, or our emails coming in over the WiFi in our house.
In recent years Dina Katabi and her students at M.I.T. CSAIL (Computer Science and Artificial Intelligence Lab) have been experimenting with processing ordinary WiFi signals down at the radio level, rather than at the bit packet level. Every phone has to do some of that, but mostly they just want to get to the bit packet, and perhaps have a little bit of quality of service information. Katabi and her students look at how timing varies very subtly and have used that to detect people, and even their emotions through detecting their breathing and heart rate, and how they are changing. Note that this does not require any sensors attached to a person–it is just detecting how the person’s physical presence changes the behavior of WiFi signals. Our future domestic robots may able to get a direct read on us in ways the people are never able to do. Whether this will count as a subjective experience for a robot will depend on how narrowly the output of the processing is drawn, and is passed on to other processing within the robot.
That was the minimal chip sets that any future domestic robot is likely to use. If the chip sets used instead consist of the full complement of communications channels that a smart phone has there will be much more richness to the Merkwelt of the robot. Using GPS, even indoors, it will roughly know its global coordinates. And it will have access to all sorts of services any smart phone uses, including the time of day, the date, the current weather and weather forecast both locally and elsewhere in the work. It will know that it is dark outside because of the time of day and year, rather than know the time of day because of how long it has been dark or light. Us humans get that sort of information in other ways. We know whether we are inside or outside not from GPS coordinates and detailed maps, but because of how it feels with light, the way the air is circulating, and how sounds reverberate. Those ways are part of our subjective experiences.
Aside: If our robots bypass the way that we experience the world with direct technological access to information it will be hard for them to understand our limitations. If they ever are consciousness they may not have much empathy for us. And should there be a horrible infrastructure destroying event (e.g., attacks on satellites, nuclear war, etc.) our robots will be left incapacitated just when we need them the most. Something to ponder as we design them.
Going back to the radio level below the bit packet level, with these additional radio frequencies, by comparing the arrival time of different signals in the many wireless domains of 5G it is possible to move from a direct Merkwelt of delays in signals to starting to understand the built environment, whether it is concrete, or wood, or whether there are large metal moving objects (trucks or buses) nearby. If the builders of our domestic robots decide to program all this in, they will have yet a weirder, to us, super-sense of the world around them.
The cameras on our robots could be chosen, easily enough, to extend the range of light that they see to ultraviolet, and/or infra red. In the later case they will be able to see where we have recently been sitting, and perhaps which way we have walked from a heat trail. That will be another weird super human sense for them to have that we don’t. But they might also tap into all the devices that are starting to populate our homes, our Amazon Echos and our Google Homes, and even our smart TVs, that can listen to us at all times. A home robot, in its terms of service which we will all agree to without reading, and through some corporate agreements might well have access to what those devices are hearing. And access to what the cameras on our smart smoke detectors are seeing. And what went on in our car right before we got home. And where we just used our electronic wallet to buy something, and perhaps what it is that we bought. These domestic robots may have a breadth of Merkwelt directly into our lives that the most controlling parent of teenage children could only imagine.
So the sensor world, the Merkwelt, may be very different for our domestic robots than for us. They will know things we can’t know, but they also may lack understanding out subjective experiences. How well they are able to relate will depend on how well their perceptual processing aligns with ours. And this is where thinking about the Merkwelt of our robots eventually gets very murky indeed.
Deep learning has been a very successful technology over the last five years. It comes from 30 years of hard work by people such as Geoff Hinton (University of Toronto and Google) and Yann LeCun (New York University and Facebook). Their work has revolutionized how well speech understanding works, and why we now have Amazon Echo, Google Home, and Apple Siri. It has also been used with very large training sets of images with labels of what is in them to train systems to themselves label images.
Here are some examples from a paper by Andrej Karpathy and Li Fei-Fei. Two networks, one trained on images and one trained on producing naturalistic English description, combined to very successfully label these three images.
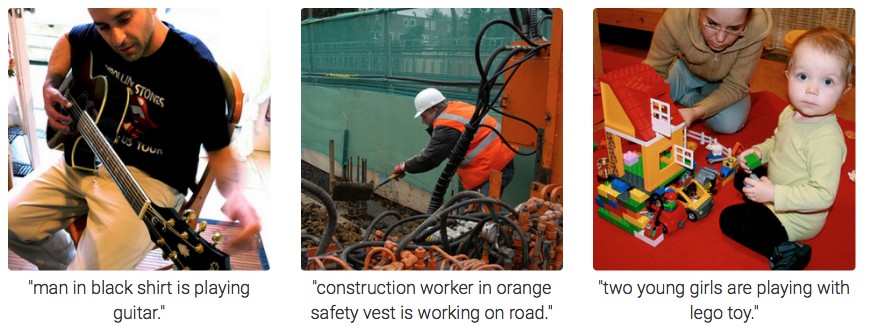
I chose these as they are particularly good examples. At their project page the authors give many more examples, some of which are not as spot on accurate as these. But just as human vision systems produce weird results sometimes, as we pointed out with the Kitaoka illusions above, so to do the deep learning vision systems.
In a paper by Anh Ngyuen, Jason Yosinski, and Jeff Clune, the authors use a deep learning trained network, trained on the same image set as the network above, and a language network that generates a label of the primary object in an image. It does great on all sorts of images that a human easily sees the same object as it claims. Their network gives a percentage certainty on its labels and the good examples all come out at over 99%.
But then they get devious and start to stress the trained network. First they use randomly generated equations to generate images when the variables are given a range of values. Some of those equations might randomly trigger the “guitar” label, say with slightly more than 0.0% likelihood–so really not something that the network believes is a guitar, just a tiny bit more than zero chance. Many images will generate tiny chances. Now they apply evolutionary computing ideas. They take two such equations and “mate” then to form lots of children equations crossing over subtrees, much as in biological systems the offspring have some DNA from each of their parents.
For instance the two expressions
and
might generate, among others, children such as
and
. Most of these children equations will get a worse score for “guitar”, but sometimes a part of each parent which tickled guitarness in the trained network might combine to give a bigger score. The evolutionary algorithm chooses better offspring like that to be parents of the next generation. Hundreds or thousands of generations later it might have evolved an equation which gets a really good score of “guitar”. The question is do those images look like guitars to us?
Here are some labels of generated images that all score better then than 99.6%.
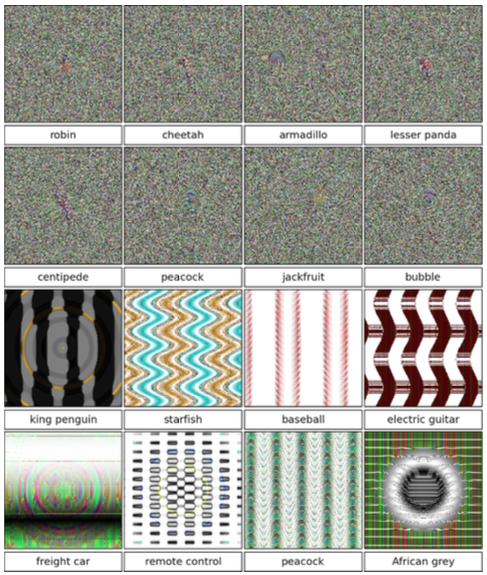
Remember, these are not purely random images that happen to trigger the labels. Vast amounts of computer time where used to breed these images to have that effect.
The top eight look like random noise to us, but these synthetic, and carefully selected images, trigger particular labels in the image classifier. The bottom ones have a little more geometric form and for some of them (e.g., “baseball”) we might see some essence of the label (i.e., some “baseballness” in things that look a bit like baseball seams to us). Below is another set of labels of generated images from their paper. The mean score across this set of labels is 99.12%, and the authors selected these results out of many, many such results as they have some essence of the label for a human vision system.
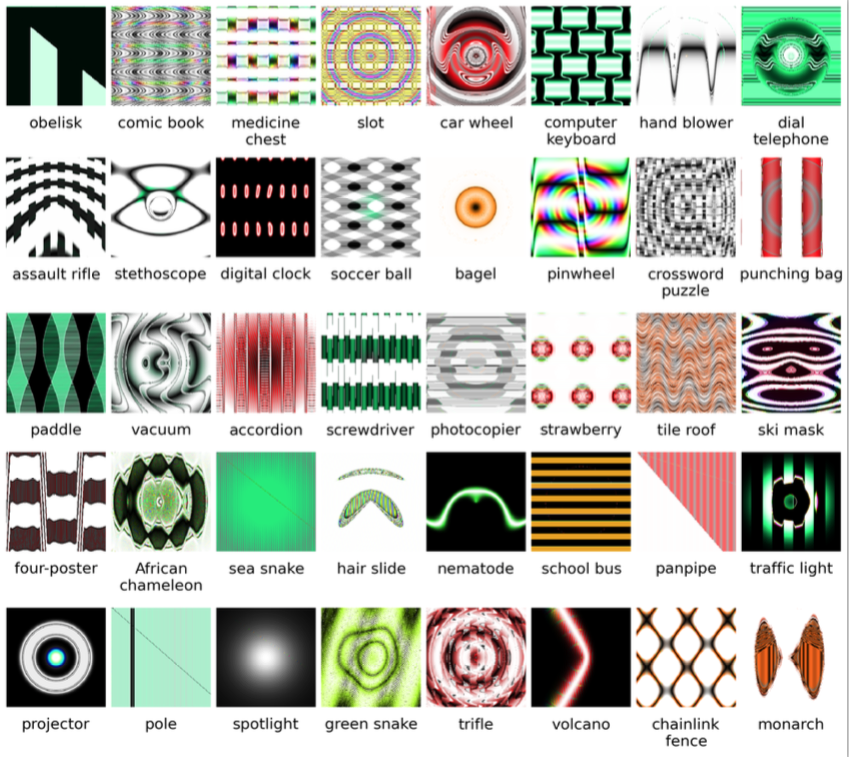
My favorite of these is “school bus”. I think all people used to seeing American school buses can get the essence of this one. In fact all of them have some essence of the label. But no competent human is going make the mistake of saying that with 99% probability these are images of the object in the label. We immediately recognize all of these as synthetic images, and know that it would be a very poor Pixar movie that tried to pass off things that looked like these images as objects that are instances of their labels.
So…all our robots are going to be subject to optical illusions that are very different from ours. They may see subtle things that we do not see, and we will see subtle things that they do not see.
The relationship between Humans and Robots
We and our domestic robots will have different Merkwelts in our shared homes. The better we understand the Merkwelt of our robot helpers, the better we will be able to have realistic expectations of what they should be able to do, and what we should delegate and entrust to them, and what aspects of our privacy we are giving up by having them around. If we build in to them a reasonable model of the human Merkwelt, the better they will be able to anticipate what we can know and will do, and so smooth our interactions.
Our robots may or may not (probably the former in the longer term) have a sense of self, subjective experiences, episodic memory blended into those subjective experiences, and even some form of consciousness, in the way that we might think our dogs have some form of consciousness. The first few generations of our domestic robots (our robot vacuum cleaners are the first generation) will be much more insect-like than mammal-like in the behavior and in their interactions with us.
Perhaps as more people start researching on how to imbue robots with subjective experiences and conscious-like experiences we will begin to have empathy with each other. Or perhaps we and our robots will always be more like an octopus and scuba diver; one is not completely comfortable in the other’s world, not as agile and aware of the details of that world, and the two of them are aware of each other, but not really engaged with each other.
Thomas Nagel, “What Is It Like to Be a Bat?”, Philosophical Review, Vol 83, No. 4, (Oct 1974), pp. 435-450.
This paper has been reprinted in a number of collections and my own copy is a 30+ year old Xeroxed version from such a collection. The original is available at JSTOR, available through university libraries, or by paying $15. However it appears that many universities around the world make it available for courses that are taught there and it is quite out in the open, from JSTOR, at many locations you can find with a search engine, e.g., here at the University of Warwick or here at the University of British Columbia.
Alexandra Horowitz, “Inside of a Dog: What Dogs See, Smell, and Know”, Scribner, New York, 2009.
Peter Godfrey-Smith, “Other Minds: the Octopus, the Sea, and the Deep Origins of Consciousness”, Farrar, Strauss and Giroux, New York, 2016.
Jakob von Uexküll, “Umwelt und Innenwelt der Tiere”, Springer, Berlin, 1921.
While checking out the origin of Merkwelt for this blog post I was surprised to see that according to Wikipedia I am one of the people .responsible for its use in English–I first used it in an AI Lab Memo 899 at M.I.T. in 1986 and then in the open literature in a paper finally published in the AI Journal in 1991 (it was written in 1987).
Lawrence Weiskrantz, “Blindsight”, Oxford University Press, Oxford, United Kingdom, 1986.
The dirt bin of the original Roomba was much too small. We had built a model two level apartment in a high bay at iRobot, and tested prototype Roombas extensively in it. We would spread dirt on different floor surfaces and make sure that Roomba could clean the floor. With the stair case we could test that it would never fall down them. When the dirt bin got full we just had the Roomba stop. But it never got close to full in a single cleaning, so we figured that people would empty the dirt bin before they left it to clean their house, and it would get all the way through a cleaning without getting full. Our tests showed it. We thought we had an appropriate model Umwelt for the Roomba. But as soon as we let Roombas out into the wild, into real people’s real apartments and houses things went wrong. The Roombas started stopping under people’s beds, and the people had to crawl under there too to retrieve them! Then it dawned on us. Most people were not cleaning under their beds before they got a Roomba. So it was incredibly dirty under there with hair balls full of dirt. The Roomba would merrily wander under a bed and get totally full of dirt! After a few cleanings this problem self corrected. There were no longer any of these incredibly dirty islands left to choke on.